1. Introduction
A systematic review (SR) attempts to collate all empirical evidence that fits pre-specified eligibility criteria to answer a specific research question. It uses explicit, systematic methods that are selected with a view to minimising bias, thus providing reliable findings from which conclusions can be drawn and decisions made (Liberati et al., 2010). A meta-analysis (MA) involves the use of statistical techniques to integrate and summarize the results of included studies. Many systematic reviews contain meta-analyses, but not all. By combining information from all relevant studies, meta-analyses can provide estimates of the effects of health care with greater precision and reduced bias than those derived from the individual studies included within a review (Liberati et al., 2010).
This guide aims to provide methodological guidance for the conduct of SR and MA of drug safety endpoints generated in completed (published or unpublished) comparative pharmacoepidemiological studies. Pharmacoepidemiological studies are defined as observational studies where individuals are exposed to drugs without a method of assignment other than by the decision of the individual or prescriber during clinical practice. The ability to conduct high quality systematic reviews and meta-analysis of pharmacoepidemiological studies is important to assess the safety of drugs from observational data that reflects everyday clinical practice. Consideration is given to the conduct of SRs and MAs from both summary data and individual-participant data.
Methods for the systematic review and meta-analysis of published and unpublished randomised controlled studies are well developed and described in the Cochrane Handbook of Systematic Reviews of Interventions (Reeves et al., 2021). As the data on safety endpoints is limited in trials at the time of approval of drugs, pharmacoepidemiological studies are important after approval. However, methods for the systematic review and meta-analysis of pharmacoepidemiological studies, in which biases and variation in risk estimates between studies take central importance, are less well developed.
The Cochrane Handbook of Systematic Reviews of Interventions (Sterne et al., 2021: Chapter 24) includes general guidance on adapting methods from randomised controlled trials (RCTs) to non-interventional studies of all types. The present stand-alone guidance is intended to fill a gap in guidance by offering a single overview and source to consult for conducting a SR and MA of completed comparative pharmacoepidemiological studies with safety endpoints. Many of the issues related to the design of individual studies within a SR are detailed in the ‘ENCePP Methods Guide’, which can be viewed as a complementary document to this guidance. These two documents will cross-reference each other.
The findings of systematic reviews and meta-analyses of pharmacoepidemiological studies should be viewed in conjunction with the totality of the evidence from randomised and non-randomised studies, pre-clinical data and pharmacological properties of the drug. It is advisable to review this evidence before embarking on a SR and MA of pharmacoepidemiological studies investigating drug safety. Such an assessment may help to pre-specify secondary hypotheses for the SR and MA, as well as providing a greater understanding of the issues and for the discussion section of the SR and MA.
2. Governance
2.1. Ethical conduct, patient and data protection
The principal ethical imperative for researchers is to obtain evidence that will contribute to protection of patients and public health. For this purpose, primary studies with as limited bias as reasonable should be obtained and the process of integration should be capable of improving the overall accuracy of the results. Thus, there is an ethical obligation for all holders of data on safety (including pharmaceutical companies and their designees, academia and medicines regulators) to contribute to the process of identification and provision of safety data. Safeguarding data confidentiality is also a key ethical consideration in public health research.
The ENCePP Code of Conduct defines requirements for the conduct of studies to maximise transparency and promote scientific independence from study sponsors throughout the research process. Annex 4 - Implementation Guidance on Sharing of ENCePP Study Data defines clear criteria for sharing data from ENCePP studies registered in the ENCePP Register as well as for the procedure for handling requests for access to data by third parties.
The fundamental issue in data protection for SR and MA are dependent on whether or not access to Individual Participant Data (IPD) for MA is sought. If IPD are provided for MA they need to remain anonymous outside of the centre that generated the original data. It should be noted that such data can be unintentionally revealed through tabulation of rare events that could lead to re-identification of a patient. There is a need, therefore, to distinguish between aggregate and individual participant level data (IPD) in terms of requirements around data protection.
2.2. Scientific standards, review and approval
Module VIII of the guideline on good pharmacovigilance practices (GVP) - Post-authorisation safety studies provides guidance for the scientific and quality standards of non-interventional post-authorisation safety studies (PASS). This includes specific instruction where the study design is a systematic review or a meta-analysis.
In some circumstances, it may be appropriate to establish a review advisory group to support the systematic review and meta-analysis. The Cochrane Handbook suggests the establishment of such groups to help reviewers outline the parameters of their review so that the end product reflects the needs of end users (Cochrane Health Promotion and Public Health Field, 2012). If established, the advisory group should provide content-related support, highlighting what needs to be included in the review.
3. Research question
3.1. Clear defintion of the research question
The objective of a systematic review and meta-analysis may be focussed on a single safety endpoint or use a wide net approach to identify and quantify a number of safety endpoints. As with SRs and MAs of RCT data, a clearly defined and focused question needs to precede any review of pharmacoepidemiological studies of safety endpoints. If the aim of the research is to seek previously unrecognised harmful effects, analysis of primary surveillance data may be more appropriate than a systematic review.
The research question should specify definitions of the:
-
Study populations (e.g. new users of a particular treatment vs. all users, newly diagnosed vs. advanced disease, prevalent vs. incident cases, time since exposure);
-
Treatment under investigation with regard to indication, treatment dose, duration and form of administration;
-
Comparison group (e.g. head-to-head comparisons between two treatments, comparison with any alternative treatment, dose-dependency etc.);
-
Safety endpoints to be studied. For example, a review might address a specific safety outcome or restricted group of related outcomes (narrow scope) or a wider group of concerns (e.g. long-term outcomes or drug interactions) (broad scope). A narrow scope review is generally recommended when there are particular safety concerns whereas broad scope reviews are suggested when the general safety of a drug is being investigated. Broad scope reviews should take into account the resources and time needed to do this comprehensively (McIntosh et al., 2004);
-
Study designs e.g. cohort studies, case-control studies, etc. For systematic reviews it is suggested that all lines of evidence are reviewed;
-
Study data sources (prospective observational studies or retrospective data from registries or database studies). Usually, all these lines of evidence are eligible for inclusion in a systematic review unless a particular safety endpoint might not be adequately reported in one source (e.g. registries) and therefore such sources might be excluded. Nonetheless, it is advisable for all sources to be gathered and when there is heterogeneity of data sources, this should be described and justified.
These components of the question also form the basis of the pre-specified eligibility criteria for the review. Some reviews may not aim at quantifying the magnitude of harm associated with a treatment but aim instead at clarifying characteristics and risk factors of the safety endpoints. These reviews might present different frameworks for the definition of the research question.
Reviews often have secondary research questions, for example, looking at secondary safety endpoints or other populations. These, often described as secondary hypotheses, need to follow a similar approach in terms of pre-specification of the population, treatment, safety endpoints, study source and design. The list of secondary research questions needs to be specified in the study protocol.
3.2. Ability to add hypotheses
As studies relevant to the original question (hypothesis) are identified during the review process, it may become clear that a new research question should be added to the original one. On some occasions, the research question might need to be modified in terms of the outcome, treatment or population definition. It is important to guard against bias in modifying research questions. It is important that all changes are noted in a protocol update and well described. The addition/revision and associated motivation should be clearly stated as such in the update and needs to consider whether the addition/revision have been influenced by results from any of the included studies.
3.3. Need for a protocol
The design of the SR and MA should be described in a protocol. Module VIII of the guideline on good pharmacovigilance practices (GVP) provides guidance on this. The protocol should describe what prior information was available and what specific information, including individual study results, motivated the research objectives of the meta-analysis. Potential anticipated study design problems and methods incorporated to address these should also be documented and/or as sensitivity analyses designed to evaluate their impact. Inclusion of aggregate data or IPD (or both) into the analysis should be specified. All predictable sensitivity analyses should be pre-defined in the study protocol and any ad-hoc sensitivity analyses need to be clearly stated in the results section. Sensitivity analyses need to be predefined in terms of population, intervention, outcome, time, study design and adjustment for important baseline variables. These types of analyses will usually address secondary rather than primary hypotheses. The protocol should be completed and finalised prior to the conduct of the research.
3.4. Registration of a protocol
The Module VIII of the guideline on good pharmacovigilance practices (GVP) - Post-authorisation safety studies recommends, for all PASS, registration of study information (including the protocol, amendments to the protocol, progress reports and final study report) in the HMA-EMA Catalogue of RWD studies. There are a number of other suitable registers, e.g. the PROSPERO database, ClinicalTrials.gov.
4. Identification and selection of studies
4.1. The 'universe' of studies
Research that is published in indexed journals is easily identifiable for SRs and MAs; however, this is only a small proportion of the universe of studies conducted.
It is known that publication bias can distort the findings of meta-analyses, be they RCTs or observational studies (Zwahlen et al., 2008; Ahmed et al., 2012). Lag time bias (where studies with unfavourable results take longer to be published) and selective outcome bias (where non-significant outcomes are omitted from publication) can also influence the results of meta-analyses (Ahmed et al., 2012). Scherer et al. (1994) showed that only about half the abstracts presented at conferences are later published in full. These effects on meta-analyses of pharmacoepidemiological studies need further investigation (Golder et al., 2010).
As searches of bibliographic databases alone may not identify all pertinent studies (Stroup et al., 2000), it is recommended that systematic search of other sources including study registers, conference abstracts, grey literature (literature not controlled by commercial publishers) and reference lists are undertaken to ensure searches are comprehensive (Sterne et al., 2021, chapter 24); Ahmed et al., 2012). Contacting investigators, MAHs/pharmaceutical industry sponsors, regulators and key data providers may also help to identify unpublished studies. For example, the Clinical Practice Research Database (CPRD) keeps a record of protocols that can be searched for these purposes. The EU PAS Register may help identify post-authorisation studies that are planned, on-going and finalised but not yet published. There is a trade-off, however, with included safety data obtained from articles with limited description of the methods used to adequately judge confounding and bias. As such they are likely to be deemed at higher risk of bias than fully reported published articles.
4.2. Data sources
A comprehensive search should be conducted (Ioannidis et al., 2006) using different bibliographic databases, hand-searching relevant journals, reference checking from identified studies, attempting to retrieve unpublished studies, contacting investigators, sponsors, data providers and regulators involved in the field of research. The following categories of data sources are suggested, and they are not mutually exclusive (e.g. CINAHL falls in “Bibliographic databases” and “Grey data”).
4.2.1. Bibliographic databases
Searches of health-related bibliographic databases are the easiest and least time-consuming way to identify an initial set of relevant reports of studies. MEDLINE and EMBASE are commonly used to identify studies with safety endpoints and can be searched electronically for key words in the title or abstract and by using standardized indexing terms, or controlled vocabulary assigned to each record.
4.2.2. Grey data – conferences, dissertations and other
Grey literature includes research with limited distribution, unpublished reports, dissertations, articles in less known (or simply non-indexed) journals, some online journals, conference abstracts, policy documents, funding agency reports, rejected or un-submitted manuscripts, non-English language articles, and technical reports (Conn et al., 2003; Lefebvre et al., 2021). There are no standards for searching the grey literature. Searches of conference proceedings, dissertations, theses and study registers should be considered. Some bibliographic databases collate conference proceedings and can give access to abstracts.
4.2.3. Investigators
Authors with multiple publications and experts in the field may be aware of unpublished or ongoing studies which include data on safety endpoints, or may have recorded data on safety endpoints not reported in the published study (McManus et al., 1998; Ioannidis et al. 2002). As safety endpoints are underreported in studies, investigators should be contacted in order to obtain safety endpoint data that may not have been reported in the original publication(s) (Zorzela et al., 2014). Research Centres / Networks in the field of interest (e.g. Cochrane Adverse Effects Methods Group or networks such as ENCePP) may direct researchers to key experts. Learned societies such as ISPE or specialists’ societies may also be useful.
4.2.4. Sponsors
Some studies conducted or sponsored by MAHs may not be published in mainstream journals hence they should be contacted for information on ongoing and unpublished observational studies. Some MAHs may make information about their studies available through their own websites.
The study sponsor could also be a government, regulatory agency, and charity or research foundation and may also be approached for unpublished studies.
4.2.5. Data providers
Contact with data providers/owners of electronic healthcare records may be helpful to identify ongoing and unpublished studies as the providers will usually have a record of studies undertaken with their data.
4.3. Search strategies
The literature search strategy is centred on key elements in the research question: population, intervention (plus acceptable comparators) and safety outcomes. It should be borne in mind that a wide definition of population may be needed as results for specific groups may not be included in searchable fields.
Two main approaches can be used, searching using index terms and searching using free-text terms. Each has its limitations, and they are best combined to maximise sensitivity (the likelihood of not missing relevant studies) and may require several iterations (Loke et al., 2007).
The MeSH (Medical Subject Headings) in MEDLINE and EMTREE in EMBASE are controlled in a vocabulary thesaurus, which consist of sets of terms naming descriptors in a hierarchical structure that permits searching at various levels of specificity within electronic databases. Subheadings are qualifiers added to MeSH subject headings to refine their meaning. Terms such as "aetiology" or "therapy", when combined with a MeSH heading, give a very precise idea of what an article covers. The Floating Subheadings (FS) field contains the 2-letter codes, such as "po" for poisoning, and is displayed following the corresponding MeSH Subject Headings. Free floating subheading are recommended as the most useful way to increase sensitivity when searching for safety endpoints in MEDLINE and EMBASE (Golder et al., 2014).
Within a bibliographic database, studies may be indexed in three different ways: (i) name of the intervention together with a subheading to denote that safety outcomes occurred (e.g. aspirin/safety endpoints), (ii) the safety endpoint together with the nature of the intervention (e.g. gastrointestinal haemorrhage/and aspirin/), or (iii) (occasionally) safety endpoint only (e.g. haemorrhage/chemically-induced).
Terms used by authors in the title and abstract of their studies can be searched on databases of electronic records using free-text terms. Two problems seriously limit the value of free-text searching. First, authors use a wide range of terms to describe safety endpoints, both in general (toxicity, side-effect, adverse-effect) and more specifically (e.g. lethargy, tiredness, malaise may be used synonymously). Therefore, as many relevant synonyms as possible should be included. Second, the free-text search does not detect safety endpoints not mentioned in the title or abstract of the study in the electronic record (even though they appear in the full report).
The syntax is specific and needs adapting for each bibliographic database and specific interface (e.g. PubMed for searching MEDLINE). It is important to spell check all search terms and control for different spellings (e.g. plasmacytoma or plasmocytoma, haematological and hematological) and to think of meaningful truncations e.g. (e.g. a PubMed search for erythrocyto* would find erythrocytosis, erythrocytotic and also erythrocytopenia). Guidance is available in the bibliographic databases. The selection of search terms is helped by clinical or epidemiological experts involved in the SR.
Date restriction should be applied if appropriate; for example, if the pharmacological treatment was not available before a certain date.
There are several commercial software tools which continue to appear to ease the effort of searches, but the elements described in this section remain valid.
Finally, the search should be described briefly in the methods section of the review. The detailed description can be made available as a web document or as an appendix in a report, where space allows.
4.4. Inconsistent use of terms to identify pharmacoepidemiological studies of harm
The assessment of whether the design of a study corresponds to the study design specified in the eligibility criteria may not be straightforward (Hartling et al., 2011). For example, the commonly used term “case-control study” covers a wide variety of types of study. The label could be applied to a study in which cases and controls are separately identified and individuals interviewed about past exposures; to case-control studies nested within cohorts in which past exposure has already been collected; and even to an analysis of a cross-sectional study in which participants are divided into cases and controls and cross-tabulated against some concurrently collected information about previous exposure. To make decisions on eligibility of studies for a SR of safety endpoints, different study types need to be distinguished from each other, and some sort of taxonomy will be needed to achieve this (Peryer et al., 2021).
For this reason, explicit study design features, and not high level study design labels must be considered when deciding which types of pharmacoepidemiological studies should be included in a review. Moreover, unlike RCTs, the design details of pharmacoepidemiological studies that are required to assess eligibility are often not described in titles or abstracts and require access to the full study report. Therefore, the search should not be limited to studies using specific design terms.
4.5. Indexing of pharmacoepidemiological studies
Methodological filters and indexing terms, such as “publication type” in MEDLINE, are useful to limit searches when conducting SRs including RCT studies. This strategy is unlikely to be helpful in pharmacoepidemiological studies because study design labels other than RCTs are not reliably indexed by bibliographic databases and are often used inconsistently by authors. Therefore, an inclusive approach is recommended (Peryer et al., 2021, chapter 19); Reeves et al., 2021, chapter 24); Golder et al., 2014). An algorithm using appropriate terms should be defined in the protocol.
4.6. Flow chart
Use of a flow diagram and text, reporting the process from identification through to the selection of studies included in SR and MA is recommended. Authors should report: unique records identified in searches; records excluded after preliminary screening (e.g. screening of titles and abstracts); reports retrieved for detailed evaluation; potentially eligible reports which were not retrievable; retrieved reports that did not meet inclusion criteria and the primary reasons for exclusion; and the studies included in the review. Below is shown a diagram from the PRISMA Statement that, despite being addressed to systematic review of interventions, is suitable to any kind of systematic review, including pharmacoepidemiological studies of harm (Liberati et al., 2009).
Flow of information through the different phases of a systematic review
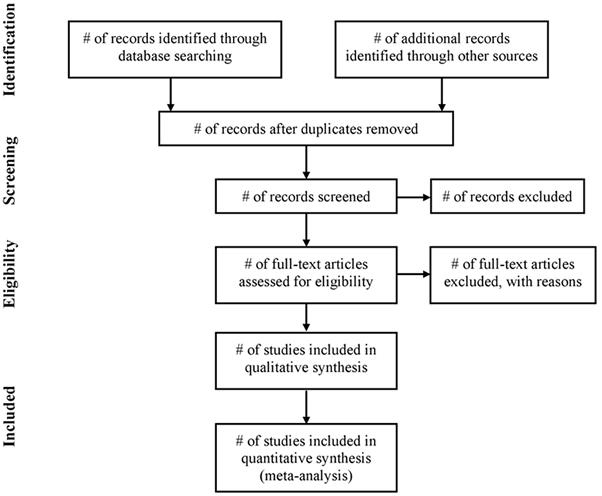
5. Assessment of selected primary research studies
5.1. Objectives of the assessment
The review of the applicability, validity and utility of selected studies can have several objectives. They may include becoming familiar with the design and data collection choices made by researchers of the studies; understanding how these choices may impact the results of the studies by examining their variability and identifying the risk of bias.
The assessment should be conducted based on the information in the source manuscript and other sources identified by two researchers either independently or in primary and review roles. It is important to keep in mind that contrary to the extraction of results, the evaluation of risk of bias has an inherent subjective component. Therefore review, discussion and consensus among assessors are essential. Piloting the process and forms is often required to adjust definitions of the domains for risk of bias. Furthermore, the forms should include space for the assessor to document the reason for the results of the risk appraisal in each domain.
5.2. Review of studies
Evaluating the validity of each of the primary research studies is one of the first steps once a study has been selected for the systematic review. Chapter 5 on Study Design of the ENCePP Guide on Methodological Standards in Pharmacoepidemiology provides an overview of the key design considerations for pharmacoepidemiological studies.
A key challenge when reviewing primary studies is the available level of details regarding the design and methods of the study, often limited by the space provided by journals. Publication of additional method details in online appendices facilitates the work of the reviewer, and reviewers should always check their existence. Researchers reporting results of the study may have followed the appropriate Strengthening the Reporting of Observational Studies in Epidemiology (STROBE) checklist (von Elm et al., 2008) and reviewers should be familiar with this guidance. The use of the ENCePP Checklist (ENCePP Checklist) for Study Protocols by researchers of primary pharmacoepidemiology studies may also promote reporting the key design and methods relevant for pharmacoepidemiology in the publication.
Additional sources of information to reviewers of primary studies, especially for more recent studies, are the protocols of primary studies registered in the HMA-EMA Catalogue of RWD studies.
5.3. Study Assessment Tools
Many tools have been developed to document and summarise the key findings regarding study design choices and validity. Some were intended for a specific review, others for a more general use by reviewers in different fields of non-experimental studies. Some were developed independently, others commissioned by agencies or research groups (e.g. US AHRQ, Cochrane).
Helpful general reviews of tools can be found in Deeks et al. (2003) and Sanderson et al. (2007). A comprehensive review of tools was conducted to assess the suitability and relevance of available tools to assess and report the design choices and validity of specifically pharmacoepidemiology studies (Neyarapally et al., 2012). The authors found that ‘the purpose and the scope of the tools varied greatly, that many available quality assessment tools do not include critical assessment elements that are specifically relevant to pharmacoepidemiological safety studies; that most tools do not distinguish between reporting elements and quality assessment attributes; and that there is a lack of reported considerations on the relative weights to assign to different domains and elements with respect to assessing the quality of these studies and in particular specific domains that are critical for pharmacoepidemiology studies’.
The Cochrane Collaboration Non-Randomized Studies Methods Working Group endorsed the use of the Newcastle Ottawa Scale 7 (NOS) (Wells et al., 2010) to assess the quality of observational studies (Sterne et al, 2021, chapter 25). In spite of its wide use, the NOS was criticised by its lack of validation (Stang, 2010), although some validation studies have been published in recent years (Oremus et al., 2012; Hartling et al., 2013). A drawback in the NOS is the lack of an “Other Bias” item. When considering the complex issues of confounding and bias the NOS star system may be misunderstood to suggest that an article is at low risk of bias even if a critical bias is present in only one domain.
Limitations have also been identified in the Cochrane Risk of Bias tool which applies only to randomised studies (Sterne et al, 2021). More comprehensive tools that cover domains to assess the risk of bias in non-randomised studies have emerged such as the ROBINS-I tool (Sterne et al., 2016). This tool attempts to cover domains comparable to those in clinical trials and does not provide a score but a broad grading of low, moderate, serious and critical risks of bias. Despite the emergence of such tools, there are still specific instances where risk of bias unique to specific outcomes or populations such as cancer or pregnancy studies require careful attention (Morales et al., 2018).
It is therefore important to keep in mind that the goal of using these tools when reviewing a primary study is to become familiar and evaluate the design choices and validity of the study in a structured, systematic, transparent and reproducible way, rather than attempt to summarize the overall quality of the study through a single score which obscures the assessment of the individual study components. Likewise including scores as a variable in the analytic model is strongly discouraged by many (Greenland and O’Rourke, 2001; da Costa et al., 2012). It is preferable to conduct stratified and sensitivity analysis based on the identified areas of risk of bias. Use of the tools as a checklist is recommended, as is the tabular or graphical display of the findings.
6. Data extraction
6.1. Aggregate data
Data extraction should be undertaken by two researchers working independently. The variables to be extracted and how they will be coded should be agreed by both researchers beforehand. At the end of each step of the process, the lead researcher identifies disagreement in the data abstracted and discusses with their co-researcher(s) until agreement on the result is obtained. Researchers should look for, note and address problems/issues which may require unforeseen changes in extraction methods. Information that does not impact the main aims of the analysis may not require duplicate extraction. This process should be made clear in the protocol and methods section.
There are different types of data to record:
a) The number of studies included and excluded, and reasons for exclusion (this may be shown with a data source flow diagram). A checklist of inclusion/exclusion criteria facilitates systematic identification of all studies fulfilling these. Systematic records should be kept of the reason(s) why each potentially eligible study from the literature search does not fulfil the criteria e.g. no control group, duplication of data included in another paper. Note that there might be more than one paper from one study, and that papers may overlap in the data they report (especially long term studies that report at intervals) – the unit of observation is the study, not the paper, and care should be taken to include study subjects only once.
b) Study-level characteristics and other data of those studies to be included in the meta-analysis. These data will enable the assessment of the risk of bias. The studies should be separated by study design, e.g. case-control, cohort, etc. This facilitates the comparison between studies.
The extraction table should be designed with variables that correspond to the critical aspects of the study design. These aspects may include:
-
purpose of the study/paper, in particular whether it was: primarily a study of efficacy of intervention, or primarily a study of safety endpoints, or an aetiological study where medication use was only one of a range of factors considered;
-
year of publication;
-
place and time period of study;
-
definition/recruitment of study population;
-
funding source;
-
the type of harm that the study had the intention and capacity to detect, e.g. length of time patients under observation after treatment;
-
how safety outcomes were reported and/or defined;
-
source of information for harm - this will then be important to decide on whether to give zero or unknown values in the tables of raw data and effect sizes;
-
exposure (intervention) definition and indication for treatment;
-
methods of analysis (e.g. multivariable logistic regression, Cox proportional hazards, etc.)
-
methods to adjust for confounding (matched sample, propensity score matching, inverse probability of treatment weighting, etc.);
-
confounders adjusted for in the analyses, as well as mentioned confounders not adjusted for;
-
proportions of missing data per analysed variable, assumed mechanism of missingness, and methods to address this (e.g. multiple imputation, full information maximum likelihood, etc.);
-
phenotyping, potential misclassification or measurement error of analysed variables, and methods to address this (e.g. regression calibration, Bayesian misclassification model, etc.).
Ensure that data relating to possible sources of heterogeneity between studies are covered explicitly, particularly study characteristics relating to sources of possible bias (e.g. retrospective recording of exposure in case-control studies, exposure-dependent attrition in cohort studies or unblinding to exposure status in recording of harm,). Formats developed elsewhere for recording study characteristics may be helpful and further practical guidance is given by the Centre for Reviews and Dissemination (university of York, UK).
c) Patient harm-related outcome and exposure data are key data. They may include patient counts for each type of harm, summary statistics of exposure time (e.g. patients-years of treatment), absolute risks, unadjusted and adjusted odds ratios/RR (with confidence intervals), hazard ratios, etc. Where harm is not dichotomous, appropriate summary measures, e.g. mean and standard deviation should be recorded in appropriate strata. Study reports may differ in the way that ordered or continuous variables are categorised. Initially it is recommended to keep as many categories as possible to allow for flexibility later on. Decisions to harmonise the categories can be made once all data have been collected and reviewed but prior to any analysis of outcomes.
It is particularly important to allow for sensitivity analyses based on the research question, e.g. including only severe adverse effects or long-term therapy. When data for a specific outcome are not found it is important to try to determine whether this is because no relevant events occurred or because the events were not considered relevant to the study. The design of tables may depend on the statistical software to be used for meta-analysis.
In designing these tables some general rules apply:
-
Safety Outcome definition. The protocol should define the scope and classification of safety outcomes to be abstracted. An attempt should be made to contact the original authors to get as much detail as possible on subgroups of safety outcomes so that consistent classification of safety outcomes can be constructed. Grouping across different categories may mask underlying specificity of safety outcomes. For cohort studies, ‘zero’, ‘presumed zero’ and ‘unknown’ entries should be distinguished. A zero should be recorded for a safety outcome if the study explicitly records zero cases. A presumed zero should be recorded if the study had the capacity to detect the safety outcome but does not mention any cases. “Unknown” should be recorded if the study did not have the capacity to detect the outcome in question or if the safety outcomes classification used in the study does not allow you to know whether a case occurred. For example, a study of safety outcomes from exposure in pregnancy may record congenital anomalies. Study A records two ‘neural tube defects’. Study B records one ‘anencephalus’ and one ‘spina bifida’. Study B is informative for ‘anencephalus’, ‘spina bifida’ and the overall category ‘neural tube defects’. Study A is informative for ‘neural tube defects’ but unknown (NOT zero) for ‘anencephalus’ and ‘spina bifida’. Aggregate categories (e.g. ‘neural tube defects’) should be included in the classification scheme because adding components (e.g. ‘anencephalus’ and ‘spina bifida’) may add up to more than the total no. of cases where one person exhibits more than one type of harm (e.g. has both ‘anencephalus’ and ‘spina bifida’). Data extraction may need to be iterative where types of harm that have not previously been identified within the classification in the protocol are mentioned in a later review of a study.
-
Definition of intervention/exposure. Data for each medication type and for each dose, including timings and quantities, should be recorded. A variable for ‘any exposure’ may also be useful.
-
Missing data (e.g. cohort members lost to follow-up, or cases/controls with unknown exposure) should be recorded.
-
Preliminary data should be recorded in as much detail as available.
-
Distinction should be made between the number of people reported with safety endpoints (within each category of safety endpoints) and the number of instances of safety endpoints where one person may be represented more than once (e.g. experiencing two subcategories of safety endpoints, or experiencing the safety endpoint more than once in the follow-up period). The number of independent persons is generally required (Ross, 2011).
Even where summary data are being extracted, if the number of cases of safety endpoints is small (rare), an attempt should be made to extract a case list with as much detail as possible about the indication, exposure itself and co-exposures, and about the diagnosis of safety endpoint.
6.2. Individual-participant data (IPD)
The use of IPD (often referred to as the original “raw” data) has a number of statistical and clinical advantages (Riley et al., 2010). Use of IPD allows centralised collection, checking, re-analysis and combination of the data. In theory, it can minimise publication and reporting biases - especially if unpublished data are obtained (Stewart et al., 2012; Ahmed et al., 2012). Although the IPD approach requires more time and resources to conduct than summary data methodology, this approach provides for: 1) increased possibilities to perform standardised and more complex statistical analyses that better reflect the true status of each patient, 2) detailed participant-level exploration of individuals’ characteristics (including inclusion and exclusion criteria, permitting consistency across studies), 3) calculation and incorporation of missing or poorly reported outcomes, 4) standardisation of subgroup categories and additional subgroup analyses not conducted in the primary studies,5) examination of the consistency of subgroup effects across studies, 6) missing data to be observed and accounted for at the individual level (Hannick et al.,2013; Riley et al.,2010; Ahmed et al.,2012), and 7) measurement error and misclassification to be observed and accounted for at the individual level (Campbell et al. 2021; de Jong et al. 2022).
The checking of data can be more extensive with IPD than with aggregate data (Stewart 1995). Areas of data quality that can be investigated include:
-
Absence of duplicate records;
-
Effects of exclusion of subjects: comparison of the data received with those available in publications; check for missing values if subject identifiers are sequential;
-
Data consistency: range checks and flag ‘outlier’ to be flagged, check of consistency of variables per subject, tabulation of numbers of subjects in each subgroup at baseline and check with publications;
-
Follow-up: duration and time points;
-
Censoring: by type;
-
Missing data, reasons for missing and auxiliary variables for imputation;
-
Internal consistencies of the data, misclassification and measurement error;
IPD can also be used to more accurately assess the appropriateness of combining studies in a meta-analysis. Considerations include:
-
Similarity of treatment regimens, including dosage and adherence to therapy;
-
Similarity of outcome measures and times of follow-up;
-
Similarity of patient characteristics;
-
Availability of covariates, including baseline measures, to define subgroups for analysis to examine subject-treatment interactions or to adjust analyses;
-
Similarity of definitions of covariates;
The advantages of IPD analyses must be weighed against the investment of time and effort involved in obtaining, understanding and analysing the complex data collected in primary studies.
6.3. Missing data
Where data required for data extraction are unavailable in the publication, but there is a reason to suppose that the authors know the information or have the data in their archive, an attempt should be made to contact the original authors to obtain the required data. Inclusion of such unpublished data should be clearly indicated and attributed. Annex IV of the ENCePP Code of Conduct may be helpful (ENCePP, 2011).
6.4. Prospective and federated meta-analyses
In a prospective meta-analysis, the meta-analysis is planned before (all) the data in the primary studies is gathered (Seidler, 2019), possibly even before some studies have started data collection . The main benefits of this approach are that 1) the meta-analysis plan is defined before results are known; 2) heterogeneity in data collection can be reduced; 3) publication bias of individual studies is avoided; 4) and selective reporting is avoided. An example is the global initiative to estimate the effects of Zika Virus during pregnancy (Zika Virus Individual Participant Data Consortium 2020). In a federated meta-analysis, no individual participant data is shared, but the analysis of primary data is performed using a centrally defined analysis plan (Gedeborg et al 2023). The aggregated results are then shared and subsequently analyses using traditional meta-analysis methods and software.
Many post-authorization safety and effectiveness studies use federated meta-analyses in of results from analyses in multiple databases and registries and can be found in the HMA-EMA Catalogue of RWD studies.
6.5. Documentation of the process of data extraction
The data extraction tables should be part of the study protocol, and any changes to the tables as well as the completed tables by each researcher, and the final tables, should be part of the audit trail of the study. If new data extraction forms have been designed, or existing forms modified to a new purpose, it is advisable to plan a pilot of the forms.
7. Statistical Analysis Plan
The Statistical Analysis Plan (SAP) should detail the planned analyses. It should adhere, broadly speaking, to the usual requirements of an SAP written for a meta-analysis of RCTs (Higgins and Green, 2011: chapter 9).
Ideally, the SAP should be written and agreed upon prior to any analyses being conducted. However, allowances should be made for post-hoc, unplanned analyses. Meta-analyses are usually conducted after some or all of the component studies are completed, and it may be unusual for a statistical analysis to be planned in ignorance of all of the results of the studies (for an exception, see section 6.4). Documentation of the state of knowledge during the planning stage may inform later interpretation of the meta-analysis or suggest appropriate sensitivity analyses.
The following points-to-consider arising from meta-analyses of RCTs will also be applicable for meta-analyses of pharmacoepidemiological studies and should be addressed in the SAP:
- Choice of study inclusion;
- Analysis of study-level and/or patient level data;
- Variation across studies in endpoint definition/derivation, patient populations;
- Multiple endpoints;
- Detection and treatment of heterogeneity;
- Model choice, including covariate adjustment;
- Inclusion of random effect(s);
- Frequentist, Bayesian and bootstrapping approaches;
- Stratification and sub-grouping including adjustments for multiplicity when necessary.
The remainder of this section focusses upon issues of particular importance for meta-analyses of pharmacoepidemiological studies.
7.1. Study Heterogeneity
It is likely that pharmacoepidemiological studies show greater inter-study variability in results compared with variability observed across RCTs. This is mainly due to the greater biases in treatment effect estimates arising from the lack of control over treatment allocation, absence of blinding, differences in length and completeness of follow-up, differences in healthcare practices, differences in database content and quality, etc. Heterogeneity may also occur as a result of differences in pharmacoepidemiological study design when data have been extracted from studies identified from a systematic review of the literature. The appropriateness of conducting a meta-analysis is questionable when the included studies show qualitatively differing results.
Methods to test for consistency should be employed (Deeks et al., 2021). Heterogeneity can be formally taken into account in a meta-analysis via the incorporation of random effects into the model. Consideration may be given to using Bayesian methods especially when the number of studies included in the meta-analysis is small. These modelling approaches allow for inter-study variability up to a certain level, but the sources of any considerable variation should be investigated further and other statistical approaches (such as the ones outlined in this section) should be considered
7.2. Study Design
The wide variety of pharmacoepidemiological study designs pose additional challenges.
Current recommendations (Stroup et al., 2000) suggest that MAs should assess the possible impact of the varying study designs via sensitivity analyses, e.g. inclusions/exclusions of certain design types, stratification/regression using design type.
Patients typically do not switch study treatments during a RCT study. However, treatment switching can and often does happen within the context of a pharmacoepidemiological study. Therefore, it is likely that analyses will need to be described in the SAP that take switching into account. There is a wealth of literature (e.g. Morden et al., 2011) that proposes a variety of statistical methods for handling this problem. These focus on RCTs but may also be applicable to pharmacoepidemiological studies.
7.3. Absolute vs. Relative Effect Sizes
Comparative pharmacoepidemiological studies will generally present data on relative effect sizes, e.g. odds ratios, hazard ratios, risk ratios, etc. However, absolute effect sizes, such as differences in incident rates, proportions, survival at a certain time-point, etc. may also be important. The absolute effect size may vary according to the population from which the relative results are derived, for instance an absolute risk reduction of 10% is not possible in a population where the risk is only 5%. For this reason, the majority of statistical approaches to meta-analyses focus on the combining of relative effect sizes. The combination of absolute effect sizes is also possible but the effect of different levels of baseline risk in the study populations should be investigated in the analysis. Instead, one may meta-analyse the relative effect measure, and subsequently use this to calculate the absolute effect measure, see The Cochrane Handbook of Systematic Reviews of Interventions (Deeks et al., 2021: Chapter 10).
7.4. Confounders
Meta-analyses of RCTs are often conducted on raw outcome data under the assumption that the elimination of selection and confounding bias at the time of randomisation will ensure an overall absence of bias in the results. This assumption is not tenable for observational data and hence it is as important to eliminate confounding in the meta-analysis as it is to do so in individual studies. The results of a single pharmacoepidemiological study with no consideration of adjustment would rarely have any validity and hence these studies should not be included when the results are meta-analysed.
There are several approaches to this: (1) to meta-analyse only the sufficiently credible adjusted results from the studies; (2) to meta-analyse only the results from studies that adjusted for the same possible confounders; or (3) to collect individual participant data with a common set of potential confounders and to repeat the adjustments for the entire dataset The first approach implies that different confounders are used by the studies included in the meta-analysis, which hampers the interpretation and introduces heterogeneity in the estimated effect. The second approach will only allow adjustment for the subset of confounders measured in all studies, which may not be sufficient to remove possible confounding. The third approach may allow for the adjustment of more possible confounders if the published studies did not adjust for possible confounders that were collected. In each case the presence or absence of important confounders should be fully documented. Such information may form the basis of a meta-regression to explain heterogeneity in the results and may be an important source of statistical heterogeneity. If there is little impact on the results this provides some reassurance that the limited common subset of confounders has little influence. Other approaches that are currently in development take uncertainty in the quality of confounding measurements into account (e.g. weighting), or account for missing data in some studies.
This problem may also be seen in terms of eligibility criteria, i.e. studies not adjusting for important confounders could be excluded and sensitivity analyses could assess how inclusion of studies with a lower level of control for confounding influences the point estimate of the meta-analysis.
7.5. Exposures and outcomes
Exposure can be difficult to measure in observational data and the first step is the verification of whether exposure has in fact occurred. Other factors include duration, patient switching, dosage changing, prior treatment (incident cohort vs. prevalent users), adherence to prescribed regimes and whether a wash-out period may be applied.
These may be viewed in the context of the eligibility criteria. They will be guided by the clinical question in so far as relevant studies can be found.
An important question in this context is whether exposures and outcomes are comparable enough for the pooling of results. It may be appropriate to perform meta-analyses of sub-sets of studies, with each one including comparable studies. However, such a decision should be carefully justified as unnecessary disaggregation of the studies will result in a loss of precision.
When multiple exposures are compared, a network meta-analysis (NMA) that simultaneously assesses direct and indirect evidence can be performed. NMA requires that the included exposures may be considered exchangeable, an assumption that is not directly testable. Instead, the studies should be qualitatively compared. A lack of exchangeability may result in inconsistent (or incoherent) effects, which is statistically testable. For observational studies this implies that adjustment is made for the same confounders, which may limit which studies can be included in the analysis alongside randomised data (Cameron et al., 2015). Individual participant data may help to improve consistency, and reduce between-study heterogeneity by adjusting for the same participant-level confounders and interaction effects and by accounting for missing data (Debray et al 2018). The use of NMA for comparative observational studies is questioned and is in its infancy.
7.6. Time considerations
Time of exposure to the product or time since exposure may be important determinants of both safety and efficacy. This should be discussed in the protocol and may affect both study selection and interpretation of the results.
Use of products may also vary over time and the validity of combining data over wide periods of calendar time should also be considered. In particular, when one treatment supersedes another the observed use may be from different times and reflect different clinical practices.
As a continuous variable, time is generally categorised on several aspects of study design and analysis, e.g. definition of past, recent or current exposure, definition of risk periods, estimated duration of prescription, lag time between two prescriptions to define treatment interruption, duration of treatment to define prevalent vs. new users, etc. Whenever these categories differ between studies and there is no access to the individual participant data, the question of the appropriateness of pooling arises. The possibility of conducting several meta-analyses based on studies with a comparable design may be considered. Differing age categories are often a problem as it is often necessary to present age-specific results or to stratify by age. When individual participant data are collected, a common definition for age groups can be applied.
7.7. Missing information and data quality
Variations in the methods for the handling of missing data pose a challenge in the meta-analysis of RCTs, and the challenges are arguably greater in the meta-analysis of pharmacoepidemiological studies. This may be explained by the wider selection of variables required to adjust for confounding or by the use of data collected in routine clinical practice independently from the research question that will be addressed, and hence without any method of prioritising important variables for complete follow-up. Loss to follow-up is also common in such data as patients elect to move to a new practice.
The amount of missing data for key variables in each study should be assessed and a decision made regarding whether the omitted data are sufficient to preclude inclusion in the analysis.
When the focus of the MA is a new safety issue that may not have been routinely recorded in the original studies it may be necessary to revert to the original study investigators for clarification.
A similar issue of general data quality exists in studies performed in routinely collected clinical datasets, where a single outcome event is recorded in different ways on multiple occasions. Formal algorithms to combine these records based on temporal proximity are sometime adopted or, preferably, the investigators may have requested clarification based on clinical notes. The procedure used in any study selected for the MA should be reported.
Variations in the handling of data quality may also pose challenges in meta-analysis. When a variable of interest is measured poorly in (some) studies, bias due to measurement error or misclassification may arise, which may propagate into the meta-analysis results. If this bias varies across studies, this may result in heterogeneity in the meta-analysis. Therefore, an attempt should be made to determine the possible presence of measurement error in each study, and a decision made regarding whether the omitted data are sufficient to preclude inclusion in the analysis. This may be difficult, as measurement error is generally poorly reported on.
When individual participant data are collected, missing data and measurement error can be accounted for. Systematic and sporadically missing data can be addressed by multiple imputations by chained equations (see, e.g., Jolani et al 2015 or Resche-Rigon and White, 2018), or a joint model (Audigier et al 2018). Measurement error and misclassification can be addressed using Bayesian methods, when either 1) gold standard measurements are available for the variable that is possibly misclassified or measured with error for some participants; or 2) multiple measurements are available per participant (Campbell et al., 2021, de Jong et al., 2023).
7.8. Study Size/Precision
In analysis of pre-existing studies, the statistical power will generally not be within the control of the researcher. Under these circumstances the ethical imperatives are different from those on investigators planning prospective research. The aim is to ensure that the uncertainty in any inference is fully characterised (e.g. via reporting of confidence limits, credible intervals, etc.) and its implications for clinical interpretation is discussed.
7.9. Individual participant data meta-analysis
When individual participant data are available, two approaches to meta-analysis are possible, the one-stage and the two-stage approach (Stewart et al., 2012) In the two-stage approach, data from each study are analysed separately in the first step, following an analysis plan defined by the meta-analysts. In the second step, standard meta-analysis methods are applied. When interaction effects are investigated, the interaction effect should be estimated separately in each study and then meta-analysed. (Fisher et al, 2011). It has been recommended to construct confidence intervals using the method of Hartung, Knapp, Sidik and Jonkman (see Inthout et al., 2014, Langan et al., 2018).
In the one-stage approach, the data are pooled, and the clustering of the data needs to be accounted for, using a mixed model (i.e., hierarchical, frailty, or mixed model) or by stratification of the intercepts (Debray et al., 2015, Legha et al., 2018) or baseline hazard (de Jong et al., 2020). Also, when investigating interaction effects, within- and between-study information needs to be separated by subtracting the mean covariate value within studies (Hua et al., 2017).
When similar modelling choices are made, including but not limited to adjusting for the same confounders, stratification of intercepts and confounder effects in the one-stage approach, using the same meta-analysis method (e.g., random effects) and the same estimation method (e.g., REML), the approaches often lead to very similar estimates (Burke et al., 2017). As the two-stage approach is noticeably more accessible, aggregate data can be included, and evidence from studies or data bases that only allow remote access can be included, performing only a two-stage meta-analysis may be sufficient. In other cases, it may be reported alongside the one-stage approach (Riley et al., 2021). The one-stage approach may be preferred when studies with small sample sizes or low event rates are included and nonlinear or interaction effects are to be estimated (Debray et al., 2015).
7.10. Sensitivity to choices of analysis methods
The results may be sensitive to the analytical method used to pool the results. Analyses are often conducted using both fixed and random effects methods. Where the likelihood of heterogeneity between observational studies is high, such as assessing risk differences, a fixed-effect method of analysis alone will rarely be justified. Analyses can also be conducted using different statistical methods when assessing statistical heterogeneity, publication bias and study level covariates (meta-regression) (Stewart et al., 2012).
In contrast with analyses of trials, the effect on the results may be analysed with different sets of covariates available in different studies and studies with different designs.
The method of analysis for conducting a meta-analysis of observational studies may differ to that from RCTs. For example, RCTs may sometimes use the number of events and sample size to generate pooled risk ratios. In contrast, this approach should be avoided when meta-analysing observational studies as it will provide only a ‘crude’ measure of association. Adjusted effect estimates from observational studies should be selected and meta-analysed.
Sensitivity analyses should be planned to assess important sources of variability that may influence the results, and should be planned at the outset. Meta-analyses of observational studies should consider all relevant effect estimates generated from included studies that may allow a better understanding of causal inference and risk of bias (Morales et al., 2018). In IPD meta-analysis, the following aspects can be explored in sensitivity analyses more easily: influence of excluded subjects, differing definitions of outcomes and standardising an outcome for the analysis, different types of censoring, duration of follow-up, time-to-event analyses, patient-treatment interactions, baseline risk and prognostic factors and scores.
8. Quality control - reproducibility of the methodology
A number of decisions must be made when carrying out a meta-analysis. The researchers should explore the effects that the use of credible alternative choices to those adopted would have on the results of the analysis. Many of these decisions may be made at the stage of development of the study protocol, including detailed description of study selection, data extraction and analysis of data to ensure methodological rigour and minimise random and systematic errors. However, some of the quality control aspects may only arise after data collection has started and require an amendment to the protocol.
A further reason for quality control is to ensure that errors and differences in interpretation in the selection, extraction, management and analysis of the data are minimised and that the steps in the SR and MA process are capable of being reproduced by independent researchers and reviewers. Typically, two independent investigators or one primary investigator and a reviewer conduct various aspects of the SR process, disagreements being resolved by discussion or with a third investigator.
A separate section may be written for the quality control aspects of the systematic review and meta-analysis, or they can be incorporated in the relevant sections of the study protocol.
8.1. Data management & statistical analysis
A robust MA will be facilitated by careful data management and statistical analysis throughout the project. It is recommended to create a single master source dataset and maintain a library of computer codes used to correct data, create new variables (aka derived data), and perform the statistical analyses. Transparency and communication with other researchers can be improved by making data (source and derived) and data cleaning & analysis codes publicly available with the project results. Both the data management and statistical analysis will usually require specialised software and personnel capable of performing these functions.
The degree of data management and statistical analysis will be much greater for IPD than summary data MA. The necessary quality control measures will clearly be different.
9. Interpretation
A focus of activity in integrating the available evidence may be to learn from the heterogeneity of designs, results and associated gaps in knowledge rather than to obtain risk estimates. Each SR and MA of pharmacoepidemiological studies will raise its own particular issues in interpretation. The following are issues which may apply to the majority of SR and MA of pharmacoepidemiological studies and should be planned for.
Methodologists, content experts and clinicians should ideally be involved in the interpretation of the results.
The results of MA usually provide a relative ratio to estimate the risk of the safety outcome. These data need to be applied to local data (using absolute event rates and exposure) to assess the public health impact of the risk on any local population.
9.1. Results compared with other sources of evidence
The results of the SR and MA need to be viewed in context with randomised controlled trials in addition to (where relevant data exist) disproportionality analyses, ecological associations, time trends and non-clinical data. Discrepancies need to be identified and explained, particularly where there is strong evidence from RCTs. It is generally considered that evidence from RCTs is more robust than evidence from pharmacoepidemiological studies. Therefore, the discussion section of the SR needs to address this issue, especially where there are discrepancies between the results of the SR of controlled pharmacoepidemiological studies and randomised evidence. Differences in aspects of the primary studies and the method of pooling for the meta-analysis of RCTs and epidemiological studies need evaluation. For example, a major difference in the meta-analyses evaluating the cardiac adverse events of cox-2 inhibitors was the use of individual-participant data in a meta-analysis of RCTs and the use of summary-level data from publications in a meta-analysis of pharmacoepidemiological studies (CNT Collaboration 2013; Varas-Lorenzo 2013). Other differences were in the number of studies identified and, for the meta-analysis based on RCTs, the ability to make both direct and indirect comparisons to check the data for accuracy before pooling and the obviously balanced nature of comparisons made. These factors may have led to the finding of a similar excess risk of cardiac events associated with celecoxib and rofecoxib (compared with placebo) in the meta-analysis of RCTs and dissimilar risks in the meta-analysis of pharmacoepidemiological studies.
9.2. Was pooling appropriate?
Whether the SR should be pooled quantitatively (meta-analysis) will depend on the clinical heterogeneity of the primary studies, the quality of the data available in the different studies and the statistical heterogeneity of the results. The decision to pool or not may have been taken early on at the protocol stage but its appropriateness may only be assessable after the SR is complete.
Reservations exist on pooling non-randomised studies where sources of bias cannot be adequately identified and adjusted for. Sources of bias can be identified when evidence for randomised and non-randomised studies is combined (Sarri et al., 2022). However, whether randomised and non-randomised studies should be formally combined rather than presented separately remains controversial.
Where substantial heterogeneity exists, techniques of meta-regression may be useful to explain and adjust the pooled estimates but usually the availability of study level covariates is limited.
Where there is substantial heterogeneity, pooling may not be appropriate, and stratum specific estimates may be more valid; the stratum may need to be reduced to that of an individual study.
9.3. Were all relevant sources of bias addressed?
The potential sources of bias in the primary studies and in the pooling of the primary studies need to be addressed and discussed in terms of how they may have influenced the results.
Bias from non-publication of studies or selective under-reporting of results should be addressed and can use visual inspection aided by funnel plots and statistical tests (Page et al., 2021).
9.4. Influence of sensitivity analyses
In view of the inherent nature of pharmacoepidemiological data harbouring unknown sources of bias, sensitivity analyses should be planned and conducted. Their influence on the results should be described, in order to assess the robustness of the results to realistic assumptions. Some sensitivity analyses cannot be planned in advance and must await the assembly of the primary studies.
The strength of these sensitivity analyses will depend on several factors, including whether summary or individual-participant data (or a mix) were available.
9.5. Limitations of the results
The SR and MA should discuss the limitations of the results and individual studies. After pooling all the available data there may still be uncertainty in the results due to large random error and imprecision due to the rarity of the safety outcome. The length of follow-up of studies may not have been sufficiently long to exclude long-term risks of the safety outcome. The scope of the studies may not reflect the majority of circumstances in which the drug is used thereby limiting the generalisability of the results of the SR and MA. Studies may not have studied particular vulnerable groups limiting the generalisability of the results to these groups.
10. Reporting requirements
10.1. Final study report
Completeness and transparency are key objectives in the reporting of a meta-analysis for the evaluation of safety. A full report describing the conduct of the analysis and sensitivity analyses is expected.
The International Society for Pharmacoepidemiology Guidelines for good pharmacoepidemiology practices (ISPE GPP) includes an ethical obligation to disseminate findings of potential scientific or public health importance and a requirement that research sponsors should be informed of study results in a manner that complies with local regulatory requirements.
The Guidance on the format and content of the final study report of non-interventional post-authorisation safety studies (PASS) provides a template for final study reports that may be applied to all non-interventional PASS, including meta-analyses and systematic reviews.
The Meta-analysis of Observational Studies in Epidemiology (MOOSE) (Stroup, 2000) group has developed a consensus statement and a list of minimum requirements for the adequate reporting of meta-analyses of observational studies in epidemiology.
When individual participant data were collected, ‘Preferred reporting items for a systematic review and meta-analysis of individual participant data: the PRISMA-IPD statement and checklist’ can be used (Stewart et al., 2015).
For network meta-analysis, ‘The PRISMA Extension Statement for Reporting of Systematic Reviews Incorporating Network Meta-analyses of Health Care Interventions: Checklist and Explanations’ can be used (Hutton et al., 2015).
The PRISMA harms checklist has been developed to facilitate the reporting of harms in systematic reviews. Additional guidance is provided in the ENCePP Code of Conduct and the IEA GEP guideline.
Authorship should conform to the guidelines established by the International Committee of Medical Journal Editors (ICJME) Recommendations ("The Uniform Requirements").
10.2. Safety reporting
The legal framework for pharmacovigilance of marketed medicinal products varies in some measure between legislative systems but many of the principles and the responsibilities placed on marketing authorisation holders and on regulatory authorities are similar. Within the EU the practical implementation of the relevant laws is explained in the Good Pharmacovigilance Practices (GVP).
Reporting of events within the individual studies will have been handled by the study organisers but if a MAH becomes aware of a meta-analysis reporting a previously unrecognised adverse drug reaction that may lead to changes in the known risk-benefit balance of a medicinal product and/or impact on public health, the relevant guidance can be found in Guideline on good pharmacovigilance practices (GVP) - Module VI – Collection, management and submission of reports of suspected adverse reactions to medicinal products.
11. Areas of uncertainty for further research
SR of observational studies is currently a less developed field than SR of RCTs. The diversity of study designs for observational data and the ubiquity of selection bias must be allowed for in the methods used for MA just as they are in the analysis of individual studies. In developing this guidance, a number of areas of uncertainty were identified that are reflected in the following list of topics which may help spur further research in this area. With regular updates to this guide, we hope these gaps will be filled and can enhance the empirical basis of this guidance.
-
Are exhaustive searches of the literature in SR of pharmacoepidemiological studies of safety outcomes as important as with RCTs?
-
Can conditions be identified in which RCTs provide similar and different results to controlled epidemiological studies? This is currently being systematically tested by a few research groups and more definite results should be available in the near future.
-
Can RCTs and controlled epidemiological studies ever be validly combined statistically?
-
Are there important differences in the results of meta-analyses of controlled epidemiological studies and RCTs when IPD are used?
-
Is MA valid when IPD is combined with study-level data e.g. use of study-level baseline characteristics?
-
How should other sources of data using different study designs be used when uncertainty remains in the validity of the results of a meta-analysis of controlled pharmacoepidemiological studies? For example, could disproportionality analyses of spontaneous adverse events or ecological studies correlating rates of adverse events with drug exposure be used to further support or refute the results of the meta-analysis?
-
What is the most effective approach to acquiring information for SR of observational study?
-
The topic of umbrella systematic reviews that implies performing a systematic review and meta-analysis of systematic reviews is an area where more experience on the field of umbrella reviews of drug effects needs to be gathered.
-
Network meta-analysis of controlled epidemiological studies has begun to be used and is an area where more experience is needed to assess its role for drug effects.
12. Update of this Annex
This Annex will be updated by regular review (frequency to be determined) and in response to new research and comments received. Please send any additional guidance documents (with the electronic link, where possible) that may be relevant to the ENCePP Secretariat to assist future updates.
13. References
Introduction (Section 1)
ENCePP. European Network of Centres of Pharmacoepidemiology and Pharmacovigilance. ENCePP Guide on Methodological Standards in Pharmacoepidemiology (Rev.3). 2014.Available from: http://www.encepp.eu/standards_and_guidances/methodologicalGuide.shtml
Liberati A, Altman DG, Tetzlaff J, Mulrow C, Gøtzsche PC, Ioannidis JP, Moher D. The PRISMA statement for reporting systematic reviews and meta-analyses of studies that evaluate health care interventions: explanation and elaboration. PLoS medicine 2009: 6(7), e1000100. doi:10.1371/journal.pmed.1000100
Reeves BC, Deeks JJ, Higgins JPT, Shea B, Tugwell P, Wells GA. Chapter 24: Including non-randomized studies on intervention effects. In: Higgins JPT, Thomas J, Chandler J, Cumpston M, Li T, Page MJ, Welch VA (editors). Cochrane Handbook for Systematic Reviews of Interventions version 6.2 (updated February 2021). Cochrane, 2021. https://training.cochrane.org/handbook/current/chapter-24
Governance (Section 2)
European Network of Centres of Pharmacoepidemiology and Pharmacovigilance. ENCePP survey of EU Member States national legal requirements on data protection. ENCePP 2013
Guidance for establishing and managing Review Advisory Groups. Cochrane Health Promotion and Public Health Field, 2012.
Guideline on good pharmacovigilance practices (GVP) Module VIII - Post-authorisation safety studies.https://www.ema.europa.eu/en/human-regulatory/post-authorisation/pharmacovigilance/good-pharmacovigilance-practices
ICMJE. International Committee of Medical Journal Editors. Recommendations for the Conduct, Reporting, Editing, and Publication of Scholarly Work in Medical Journals. Updated December 2013. Available from: http://www.icmje.org/recommendations/
Research question (Section 3)
ClinicalTrials.gov. 2014. Available from: https://clinicaltrials.gov/ct2/manage-recs/how-register
Guideline on good pharmacovigilance practices (GVP) Module VIII - Post-authorisation safety studies. Available from: https://www.ema.europa.eu/en/human-regulatory/post-authorisation/pharmacovigilance/good-pharmacovigilance-practices
McIntosh H.M, Woolacott N.F, Bagnall A.M . Assessing harmful effects in systematic Reviews BMC Medical Research Methodology 2004, 4:19 doi:10.1186/1471-2288-4-19.
PROSPERO. 2014. Available from: http://www.crd.york.ac.uk/PROSPERO/
Identification and selection of studies (Section 4)
Ahmed I, Sutton AJ, Riley RD. Assessment of publication bias, selection bias, and unavailable data in meta-analyses using individual participant data: a database survey. BMJ 2012;344:d7762.
Conn VS, Valentine JC, Cooper HM, Rantz MJ. Grey literature in meta-analyses. Nursing Research 2003; 52(4): 256–61.
Golder S, Loke YK, Bland M. Unpublished data can be of value in systematic reviews of adverse effects: methodological overview. Journal of clinical epidemiology 2010; 63(10): 1071–81.
Golder S, Loke YK, Zorzela L. Comparison of search strategies in systematic reviews of adverse effects to other systematic reviews. Health Info Libr J 2014; 31:92–105.
Hartling L, Bond K, Santaguida PL, Viswanathan M, Dryden DM. Testing a tool for the classification of study designs in systematic reviews of interventions and exposures showed moderate reliability and low accuracy. Journal of Clinical Epidemiology 2011; 64: 861–71.
Higgins JP, Ramsay C, Reeves BC, Deeks JJ, Shea B, Valentine JC, Wells G. Issues relating to study design and risk of bias when including non-randomized studies in systematic reviews on the effects of interventions. Research Synthesis Methods 2013; 4(1): 12–25.
Ioannidis JP, Chew P, Lau J. Standardized retrieval of side effects data for meta-analysis of safety outcomes. A feasibility study in acute sinusitis. J Clin Epidemiol 2002;55:619-26.
Ioannidis JP, Mulrow CD, Goodman SN. Adverse events: the more you search, the more you find. Annals of Internal Medicine 2006; 144: 298–300.
Lefebvre C, Glanville J, Briscoe S, Littlewood A, Marshall C, Metzendorf M-I, Noel-Storr A, Rader T, Shokraneh F, Thomas J, Wieland LS. Chapter 4: Searching for and selecting studies. In: Higgins JPT, Thomas J, Chandler J, Cumpston M, Li T, Page MJ, Welch VA (editors). Cochrane Handbook for Systematic Reviews of Interventions version 6.2 (updated February 2021). Cochrane, 2021. Available from www.training.cochrane.org/handbook.
Liberati A, Altman DG, Tetzlaff J, Mulrow C, Gøtzsche PC, Ioannidis JP, Moher D. The PRISMA statement for reporting systematic reviews and meta-analyses of studies that evaluate health care interventions: explanation and elaboration. PLoS medicine 2009: 6(7), e1000100. doi:10.1371/journal.pmed.1000100
Loke YK, Price D, Herxheimer A. Systematic reviews of adverse effects: framework for a structured approach. BMC medical research methodology 2007: 7; 32.
McManus RJ, Wilson S, Delaney BC, et al. Review of the usefulness of contacting other experts when conducting a literature search for systematic reviews. BMJ. 1998;317:1562-1563.
Peryer G, Golder S, Junqueira D, Vohra S, Loke YK. Chapter 19: Adverse effects. In: Higgins JPT, Thomas J, Chandler J, Cumpston M, Li T, Page MJ, Welch VA (editors). Cochrane Handbook for Systematic Reviews of Interventions version 6.2 (updated February 2021). Cochrane, 2021. Available from www.training.cochrane.org/handbook.
Reeves BC, Deeks JJ, Higgins JPT, Shea B, Tugwell P, Wells GA. Chapter 24: Including non-randomized studies on intervention effects. In: Higgins JPT, Thomas J, Chandler J, Cumpston M, Li T, Page MJ, Welch VA (editors). Cochrane Handbook for Systematic Reviews of Interventions version 6.2 (updated February 2021). Cochrane, 2021. Available from www.training.cochrane.org/handbook.Scherer RW, Dickersin K, Langenberg P.1994. Full publication of results initially presented in abstracts. A meta-analysis. JAMA 1994;272: 158–62.
Stroup DF, Berlin JA, Morton SC, Olkin I, Williamson GD, Rennie D, Moher D, Becker BJ, Sipe TA, Thacker SB. Meta-analysis of observational studies in epidemiology: a proposal for reporting. Meta-analysis Of Observational Studies in Epidemiology (MOOSE) group. JAMA 2000;283(15):2008-2012.
Centre for Reviews and Dissemination, and Jo Akers. Systematic reviews: CRD's guidance for undertaking reviews in health care. Centre for Reviews and Dissemination, 2009. University of York.
Zorzela L, Golder S, Liu Y, Pilkington K, Hartling L, Joffe A, Loke Y, Vohra S. Quality of reporting in systematic reviews of adverse events: systematic review. http://www.bmj.com/content/348/bmj.f7668/rapid-responses BMJ 2014; 348:g1394
Zwahlen M, Renehan A, Egger M. Meta-analysis in medical research: potentials and limitations. Urologic Oncology: Seminars and Original Investigations 2008;26:320-329.
The EU PAS Register (ENCePP E-Register of Studies)
MEDLINE (www.nlm.nih.gov/pubs/factsheets/medline.html)
https://www.nlm.nih.gov/bsd/disted/pubmedtutorial/cover.html (the tutorial)
EMBASE (www.elsevier.com/online-tools/embase)
Assessment of selected primary research studies (Section 5)
Greenland S., O'Rourke K. On the bias produced by quality scores in meta-analysis, and a hierarchical view of proposed solutions. Biostatistics. 2001 Dec;2(4):463-71.
da Costa BR1, Hilfiker R, Egger M. PEDro's bias: summary quality scores should not be used in meta-analysis. J Clin Epidemiol. 2013 Jan;66(1):75-7. doi: 10.1016/j.jclinepi.2012.08.003.
von Elm E, Altman DG, Egger M, Pocock SJ, Gøtzsche PC, Vandenbroucke JP; STROBE Initiative. The Strengthening the Reporting of Observational Studies in Epidemiology (STROBE) statement: guidelines for reporting observational studies. J Clin Epidemiol. 2008 Apr; 61(4):344-9.
Deeks JJ1, Dinnes J, D'Amico R, Sowden AJ, Sakarovitch C, Song F, Petticrew M, Altman DG; International Stroke Trial Collaborative Group; European Carotid Surgery Trial Collaborative Group. Evaluating non-randomised intervention studies. Health Technol Assess. 2003;7(27):iii-x, 1-173.
Sanderson S1, Tatt ID, Higgins JP. Tools for assessing quality and susceptibility to bias in observational studies in epidemiology: a systematic review and annotated bibliography. Int J Epidemiol. 2007 Jun;36(3):666-76
Neyarapally GA et al. Review of quality assessment tools for the evaluation of pharmacoepidemiological safety studies. BMJ Open 2012;2:e001362. doi:10.1136/bmjopen-2012 001362
Wells GA, Shea B, O'Connell D, Peterson J, Welch V, Losos M, Tugwell P. The Newcastle–Ottawa Scale (NOS) for assessing the quailty of nonrandomised studies in meta-analyses. Ottawa, Ontario: Ottawa Health Research Institute 2010.
Sterne JAC, Hernán MA, McAleenan A, Reeves BC, Higgins JPT. Chapter 25: Assessing risk of bias in a non-randomized study. In: Higgins JPT, Thomas J, Chandler J, Cumpston M, Li T, Page MJ, Welch VA (editors). Cochrane Handbook for Systematic Reviews of Interventions version 6.2 (updated February 2021). Cochrane, 2021. Available from www.training.cochrane.org/handbook.
Stang A. Critical evaluation of the Newcastle-Ottawa scale for the assessment of the quality of nonrandomized studies in meta-analyses. European Journal of Epidemiology September 2010, Volume 25, Issue 9, pp 603-605
Oremus M, Oremus C, Hall GB, McKinnon MC. Inter-rater and test-retest reliability of quality assessments by novice student raters using the Jadad and Newcastle-Ottawa Scales. BMJ Open. 2012;2(4).
Sterne JAC, Hernán MA, Reeves BC, Savović J, Berkman ND, Viswanathan M, Henry D, Altman DG, Ansari MT, Boutron I, Carpenter JR, Chan AW, Churchill R, Deeks JJ, Hróbjartsson A, Kirkham J, Jüni P, Loke YK, Pigott TD, Ramsay CR, Regidor D, Rothstein HR, Sandhu L, Santaguida PL, Schünemann HJ, Shea B, Shrier I, Tugwell P, Turner L, Valentine JC, Waddington H, Waters E, Wells GA, Whiting PF, Higgins JPT. ROBINS-I: a tool for assessing risk of bias in non-randomized studies of interventions. BMJ 2016;355:i4919
Morales DR, Slattery J, Evans S, Kurz X. Antidepressant use during pregnancy and risk of autism spectrum disorder and attention deficit hyperactivity disorder: systematic review of observational studies and methodological considerations. BMC Med. 2018;16(1):6.
Hartling L, Milne A, Hamm MP, et al. Testing the Newcastle Ottawa Scale showed low reliability between individual reviewers. J Clin Epidemiol. 2013;66(9):982-993.
Viswanathan M, Berkman ND. Development of the RTI item bank on risk of bias and precision of observational studies. Rockville (MD): Agency for Healthcare Research and Quality; 2011.
Viswanathan M, Berkman ND. Development of the RTI item bank on risk of bias and precision of observational studies. J Clin Epidemiol. 2012;65(2):163-178.
Margulis AV, Pladevall M, Riera-Guardia N, Varas-Lorenzo C, Hazell L, Perez-Gutthann S. Quality Assessment of Observational Studies in a Drug-Safety Systematic Review--Comparison of Two Tools: the Newcastle-Ottawa Scale and the RTI Item Bank. Clinical Epidemiology 2014; 359-368.
Data extraction (Section 6)
Ahmed I, Sutton AJ, Riley RD. Assessment of publication bias, selection bias, and unavailable data in meta-analyses using individual participant data: a database survey. BMJ 2012;344:d7762.
Campbell H, de Jong VMT, Maxwell L, Jaenisch T, Debray TPA, Gustafson P. Measurement error in meta-analysis (MEMA)—A Bayesian framework for continuous outcome data subject to non-differential measurement error. Research Synthesis Methods. 2021;12(6):796–815. Available from: https://doi.org/10.1002/jrsm.1515
de Jong VMT, Campbell H, Maxwell L, Jaenisch T, Gustafson P, Debray TPA. Adjusting for misclassification of an exposure in an individual participant data meta-analysis. Research Synthesis Methods. 2023;14(2):193–210. Available from: https://onlinelibrary.wiley.com/doi/abs/10.1002/jrsm.1606European Network of Centres of Pharmacoepidemiology and Pharmacovigilance. ENCePP Code of Conduct – Implementation Guidance for Sharing of ENCePP Study Data . ENCePP 2011.
Gedeborg R, Igl W, Svennblad B, Wilén P, Delcoigne B, Michaëlsson K, et al. Federated analyses of multiple data sources in drug safety studies. Pharmacoepidemiology and Drug Safety. 2023;32(3):279–86. Available from: https://onlinelibrary.wiley.com/doi/abs/10.1002/pds.5587
Hannink G, Gooszen HG, van Laarhoven CJHM, Rovers MM. A systematic review of individual patient data meta-analyses on surgical interventions. Systematic Reviews 2013;2:52.
Jolani S, Debray TPA, Koffijberg H, Buuren S, Moons KGM. Imputation of systematically missing predictors in an individual participant data meta-analysis: a generalized approach using MICE. Statistics in Medicine. 2015;34(11):1841–63.
Resche-Rigon M, White IR. Multiple imputation by chained equations for systematically and sporadically missing multilevel data. Stat Methods Med Res. 2018 Jun;27(6):1634–49.
Riley RD, Lambert PC, Abo-Zaid G. Meta-analysis of individual participant data: rationale, conduct, and reporting. BMJ 2010;340:c221
Seidler AL, Hunter KE, Cheyne S, Ghersi D, Berlin JA, Askie L. A guide to prospective meta-analysis. BMJ. 2019 Oct 9;367:l5342. Available from: https://doi.org/10.1136/bmj.l5342
Stewart GB, Altman DG, Askie LM, Duley L, Simmonds MC, Stewart LA. Statistical analysis of individual participant data meta-analyses: a comparison of methods and recommendations for practice. PLoS ONE 2012;7(10):e46042.
Zika Virus Individual Participant Data Consortium. The Zika Virus Individual Participant Data Consortium: A Global Initiative to Estimate the Effects of Exposure to Zika Virus during Pregnancy on Adverse Fetal, Infant, and Child Health Outcomes. Tropical Medicine and Infectious Disease. 2020 Dec;5(4):152. Available from: https://doi.org/10.3390/tropicalmed5040152
Statistical Analysis Plan (Section 7)
Audigier V, White IR, Jolani S, Debray TPA, Quartagno M, Carpenter J, et al. Multiple Imputation for Multilevel Data with Continuous and Binary Variables. Statist Sci. 2018 May;33(2):160–83. Available from: https://doi.org/10.1214/18-STS646
Burke DL, Ensor J, Riley RD. Meta-analysis using individual participant data: one-stage and two-stage approaches, and why they may differ. Statistics in Medicine. 2017;36(5):855–75.
Campbell H, de Jong VMT, Maxwell L, Jaenisch T, Debray TPA, Gustafson P. Measurement error in meta-analysis (MEMA)—A Bayesian framework for continuous outcome data subject to non-differential measurement error. Research Synthesis Methods. 2021;12(6):796–815. Available from: https://doi.org/10.1002/jrsm.1515
Cameron C, Fireman B, Hutton B, Clifford T, Coyle D, Wells G, et al. Network meta-analysis incorporating randomized controlled trials and non-randomized comparative cohort studies for assessing the safety and effectiveness of medical treatments: challenges and opportunities. Systematic Reviews. 2015 Nov 5;4(1):147. Available from: https://doi.org/10.1186/s13643-015-0133-0
Debray TP, Schuit E, Efthimiou O, Reitsma JB, Ioannidis JP, Salanti G, et al. An overview of methods for network meta-analysis using individual participant data: when do benefits arise? Stat Methods Med Res. 2018 May 1;27(5):1351–64. Available from: https://doi.org/10.1177/0962280216660741
Debray TPA, Moons KGM, van Valkenhoef G, Efthimiou O, Hummel N, Groenwold RHH, et al. Get real in individual participant data (IPD) meta-analysis: a review of the methodology. Res Synth Methods. 2015 Dec;6(4):293–309.
Debray TPA, Moons KGM, Abo-Zaid GMA, Koffijberg H, Riley RD. Individual Participant Data Meta-Analysis for a Binary Outcome: One-Stage or Two-Stage? Hernandez AV, editor. PLoS ONE. 2013 Apr 9;8(4):e60650. Available from: https://dx.plos.org/10.1371/journal.pone.0060650
Deeks JJ, Higgins JPT, Altman DG (editors). Chapter 10: Analysing data and undertaking meta-analyses. In: Higgins JPT, Thomas J, Chandler J, Cumpston M, Li T, Page MJ, Welch VA (editors). Cochrane Handbook for Systematic Reviews of Interventions version 6.2 (updated February 2021). Cochrane, 2021. Available from www.training.cochrane.org/handbook.
de Jong VMT, Campbell H, Maxwell L, Jaenisch T, Gustafson P, Debray TPA. Adjusting for misclassification of an exposure in an individual participant data meta-analysis. Research Synthesis Methods. 2023;14(2):193–210. Available from: https://onlinelibrary.wiley.com/doi/abs/10.1002/jrsm.1606
de Jong VMT, Moons KGM, Riley RD, Smith CT, Marson AG, Eijkemans MJC, et al. Individual participant data meta-analysis of intervention studies with time-to-event outcomes: A review of the methodology and an applied example. Research Synthesis Methods. 2020;11(2):148–68. Available from: https://doi.org/10.1002/jrsm.1384
Fisher DJ, Copas AJ, Tierney JF, Parmar MKB. A critical review of methods for the assessment of patient-level interactions in individual participant data meta-analysis of randomized trials, and guidance for practitioners. Journal of Clinical Epidemiology. 2011 Sep 1;64(9):949–67. Available from: http://www.sciencedirect.com/science/article/pii/S0895435610004294
Hua H, Burke DL, Crowther MJ, Ensor J, Tudur Smith C, Riley RD. One-stage individual participant data meta-analysis models: estimation of treatment-covariate interactions must avoid ecological bias by separating out within-trial and across-trial information. Statistics in Medicine. 2017;36(5):772–89.
IntHout J, Ioannidis JPA, Borm GF. The Hartung-Knapp-Sidik-Jonkman method for random effects meta-analysis is straightforward and considerably outperforms the standard DerSimonian-Laird method. BMC Medical Research Methodology. 2014;14(1):25.
Langan D, Higgins JPT, Jackson D, Bowden J, Veroniki AA, Kontopantelis E, et al. A comparison of heterogeneity variance estimators in simulated random-effects meta-analyses. Res Synth Methods. 2018 Aug 1;10(1):83--98.
Legha A, Riley RD, Ensor J, Snell KI, Morris TP, Burke DL. Individual participant data meta-analysis of continuous outcomes: A comparison of approaches for specifying and estimating one-stage models. Statistics in Medicine. 2018;Morales DR, Slattery J, Evans S, Kurz X. Antidepressant use during pregnancy and risk of autism spectrum disorder and attention deficit hyperactivity disorder: systematic review of observational studies and ethodological considerations. BMC Med. 2018;16(1):6
Morden JP, Lambert PC, Latimer NR, Abrams KR, Wailoo AJ. Assessing methods for dealing with treatment switching in randomised controlled trials: a simulation study. BMC Med Res Methodol. 2011; 11.
Riley RD, Steyerberg EW. Meta-analysis of a binary outcome using individual participant data and aggregate data. Res Synth Method. 2010 Jan;1(1):2–19. Available from: http://doi.wiley.com/10.1002/jrsm.4
Riley RD, Burke DL, Morris T. One-stage versus Two-stage Approach to IPD Meta-Analysis. In: Individual Participant Data Meta-Analysis. John Wiley & Sons, Ltd; 2021. p. 199–217. Available from: https://onlinelibrary.wiley.com/doi/abs/10.1002/9781119333784.ch8
Stroup DF, Berlin JA, Morton SC, Olkin I, Williamson GD, Rennie D, Moher D, Becker BJ, Sipe TA, Thacker SB. Meta-analysis of observational studies in epidemiology: a proposal for reporting. Meta-analysis Of Observational Studies in Epidemiology (MOOSE) group. JAMA 2000;283(15):2008-2012.
Stewart GB, Altman DG, Askie LM, Duley L, Simmonds MC, Stewart LA. Statistical analysis of individual participant data meta-analyses: a comparison of methods and recommendations for practice. PLoS One. 2012;7(10):e46042.
Interpretation (Section 9)
Coxib and traditional NSAID Trialists' (CNT) Collaboration, Bhala N, Emberson J, Merhi A, Abramson S, Arber N, Baron JA, Bombardier C, Cannon C, Farkouh ME, FitzGerald GA, Goss P, Halls H, Hawk E, Hawkey C, Hennekens C, Hochberg M, Holland LE, Kearney PM, Laine L, Lanas A, Lance P, Laupacis A, Oates J, Patrono C, Schnitzer TJ, Solomon S, Tugwell P, Wilson K, Wittes J, Baigent C. Vascular and upper gastrointestinal effects of non-steroidal anti-inflammatory drugs: meta-analyses of individual participant data from randomised trials. Lancet. 2013;382:769-79
Page MJ, Higgins JPT, Sterne JAC. Chapter 13: Assessing risk of bias due to missing results in a synthesis. In: Higgins JPT, Thomas J, Chandler J, Cumpston M, Li T, Page MJ, Welch VA (editors). Cochrane Handbook for Systematic Reviews of Interventions version 6.2 (updated February 2021). Cochrane, 2021.
Sarri G, Patorno E, Yuan H, Guo JJ, Bennett D, Wen X, et al. Framework for the synthesis of non-randomised studies and randomised controlled trials: a guidance on conducting a systematic review and meta-analysis for healthcare decision making. BMJ Evid Based Med. 2022 Apr;27(2):109–19.
Varas-Lorenzo C, Riera-Guardia N, Calingaert B, Castellsague J, Salvo F, Nicotra F, Sturkenboom M, Perez-Gutthann S. Myocardial infarction and individual nonsteroidal anti-inflammatory drugs meta-analysis of observational studies. Pharmacoepidemiol Drug Saf. 2013;22:559-70.
Reporting requirements (Section 10)
European Network of Centres of Pharmacoepidemiology and Pharmacovigilance. ENCePP Code of Conduct – Implementation Guidance for Sharing of ENCePP Study Data. ENCePP 2011.
Guideline on good pharmacovigilance practices (GVP) Module VI – Management and reporting of adverse reactions to medicinal products (Rev 1). https://www.ema.europa.eu/en/human-regulatory/post-authorisation/pharmacovigilance/good-pharmacovigilance-practices
IEA GEP for Proper Conduct in Epidemiologic Research, November, 2007.
https://ieaweb.org/IEAWeb/Content/IEA_Publications.aspx
ICMJE. Recommendations for the Conduct, Reporting, Editing, and Publication of Scholarly Work in Medical Journals. Updated December 2013.
The PRISMA Extension Statement for Reporting of Systematic Reviews Incorporating Network Meta-analyses of Health Care Interventions: Checklist and Explanations. Ann Intern Med. 2015 Jun 2;162(11):777–84. Available from: https://www.acpjournals.org/doi/full/10.7326/M14-2385
Stewart LA, Clarke M, Rovers M, Riley RD, Simmonds M, Stewart G, et al. Preferred reporting items for a systematic review and meta-analysis of individual participant data: the PRISMA-IPD statement. Jama. 2015;313(16):1657–65.
Stroup DF, Berlin JA, Morton SC, Olkin I, Williamson GD, Rennie D, Moher D, Becker BJ, Sipe TA, Thacker SB. Meta-analysis of observational studies in epidemiology: a proposal for reporting. Meta-analysis Of Observational Studies in Epidemiology (MOOSE) group. JAMA 2000;283(15):2008-2012.
Zorzela L, Loke YK, Ioannidis JP, Golder S, Santaguida P, Altman DG, et al. PRISMA harms checklist: improving harms reporting in systematic reviews. BMJ. 2016 Feb 1;i157. Available from: https://www.bmj.com/lookup/doi/10.1136/bmj.i157