1. General considerations
Planning a pregnancy may lead to discontinuation of non-essential medicines before conception (Pregnancy as a major determinant for discontinuation of antidepressants: an analysis of data from The Health Improvement Network, J Clin Psychiatry. 2011;72(7):979-85). However, many pregnancies are unplanned, and not all medicine use can be discontinued without harm to the pregnant parent and, directly or indirectly, the foetus. Increasing average reproductive age in the western societies (Women in the EU are having their first child later - Eurostat) and the growing prevalence of chronic diseases in pregnant populations (Prevalence of maternal chronic diseases during pregnancy - a nationwide population based study from 1989 to 2013, Acta Obstet Gynecol Scand. 2016;95(11):1295-1304) underscore the importance of evidence generation on medicine use in pregnancy. In particular, large, well-conducted studies suggesting ‘null’ associations are important to ensure availability of treatment options for pregnant people.
Medicines vary with respect to their bioavailability during pregnancy, and their ability to cross the placental barrier (Pregnancy-Associated Changes in Pharmacokinetics: A Systematic Review, PLoS Med. 2016;13(11):e1002160). Clinically, decisions regarding safety in pregnancy depend on the balance between the benefits of treatment for the mother and the potential risks to the unborn child. There is often uncertainty about these risks because pregnant people rarely participate in pre-authorisation trials. Evidence regarding safety of medicines in pregnancy comes primarily from post-authorisation observational studies and prior to that, from animal studies. Increasingly, post-authorisation studies are relying on routinely collected data from large healthcare or claims databases. For these data sources to yield valid results, multidisciplinary expert knowledge and tailored methodological approaches are required (Use of real-world evidence from healthcare utilization data to evaluate drug safety during pregnancy, Pharmacoepidemiol Drug Saf. 2019;28(7):906-22).
Impact of medicine use in pregnancy differs from the impact of use in breastfeeding. Use of many teratogenic medicines may be incompatible with motherhood. When it comes to safety of a medicine during breastfeeding, a benefit-risk decision needs to be made regarding whether or not to breastfeed; carefully time medication intake vis-a-vis breastfeeding the infant; or discontinue it. It is not incompatible with motherhood.
This Annex summarises methodological aspects relevant for study design and interpretation in the evaluation of medicines during pregnancy and breastfeeding, with some focus on safety. Pharmacoepidemiological studies in routinely collected clinical health records and claims data are discussed, as well as aspects relevant to these studies in other data sources. The safety of medicines during breastfeeding is a relatively recent topic and is discussed at the end of this Annex.
2. Study designs and populations
As with any pharmacoepidemiological studies, thoughtful, evidence- and hypothesis-driven selection of outcomes is encouraged. In pregnancy research, outcomes of interest may vary by timing of exposure in pregnancy and hence, a good understanding of embryofoetal development is crucial. The basics of reproductive epidemiology and birth outcomes as well as basics of pregnancy-specific pharmacokinetics are covered systematically in the respective textbooks and reviews, see for example the chapter on reproductive epidemiology by Weinberg & Wilcox in Modern Epidemiology 4th ed. (T. Lash, T.J. VanderWeele, S. Haneuse, K. Rothman. Wolters Kluwer, 2020). Pregnancy is a unit with implications for health of two or more individuals. Reproductive epidemiology studies usually assess a set of pregnancy, maternal, embryo-foetal, birth, and/or neonatal outcomes. The unit of observation therefore could be a pregnant parent, a pregnancy, an embryo/foetus, and/or a still- or liveborn child. Pregnancy and birth outcomes are on a continuum and are dependent on each other. For example, a pregnancy that ends in an early loss typically will not be included in a study of evaluating the risk of malformations, or a well-controlled disease in the mother may lead to a healthier child. Teratogenic drugs generally produce a specific pattern of abnormalities, or a single malformation during a sensitive period of gestation. To detect a potential teratogen, all types of malformations may need to be assessed initially, bearing in mind not all malformations are immediately diagnosed at birth or even in the first year of life. In addition to malformations, a teratogen may manifest in other adverse reproductive outcomes including reduced fertility, pregnancy loss. This needs to be considered when choosing a study design to evaluate pregnancy outcomes.
To design a valid study that takes adequate account of this continuum of development, ideally, the multidisciplinary study team should include (pharmaco)epidemiologists, biostatisticians, perinatologists/embryologists, reproductive toxicologists, specialists in the maternal disease studied, pharmacokinetics, and in obstetrics and, if longer term neurodevelopmental outcomes are being considered, paediatricians and /or child psychologists/psychiatrists (depending on the outcomes under investigation). The study team needs to be able to determine, for example, during which time in gestation certain birth defects arise, which birth defects occur from interference within the same embryonic tissue and hence, potentially may be treated as ‘one outcome’, as well as what the impact of 2nd or 3rd trimester exposures may be on infant growth and development and when in a child’s life such impact can be assessed.
Pregnancy research is unique in that, while exposure occurs in the mother and, through the mother, in the child, outcomes can occur at the level of pregnant individual (e.g., death), pregnancy (e.g., preeclampsia, urinary tract infection), or foetus/infant (e.g., small for gestational age, congenital malformations; one infant can have multiple congenital malformations). One person can contribute several pregnancies to a given study; multi-foetal pregnancies result in more than one neonate. The unit of analysis needs to be specified for each analysis (see Table 1). Strategies to handle the potential mismatch between the number of exposed people and the number of observations that can develop the outcome include conducting analyses at the most granular level (e.g., including twins as separate units), assigning the worst outcome to multi-foetal pregnancies (e.g., if at least one foetus has a malformation, the pregnancy is considered to have resulted in a malformation) and including one pregnancy per person or one foetus per pregnancy.
Family clusters share the environment and parental characteristics (including genes, demographics, lifestyles, medical conditions, etc.) and create the potential for within-family correlation. This violates the independence assumption underlying commonly used statistical models. Problems arising from ignoring the clustering can be large when clustering is substantial (Regression models for clustered binary responses: implications of ignoring the intracluster correlation in an analysis of perinatal mortality in twin gestations, Ann Epidemiol. 2005;15(4):293-301). While selecting one pregnancy or baby per cluster eliminates the correlation, it also reduces study size and excludes potentially relevant information. An alternative is to take the clustering into account in the analyses of the data e.g., by multilevel modelling. On the other hand, family clusters allow controlling for family factors using family-specific methods, such as the sibling design (Regression models for clustered binary responses: implications of ignoring the intracluster correlation in an analysis of perinatal mortality in twin gestations, Ann Epidemiol. 2005;15(4):293-301; On Sibling Designs, Epidemiology 2013;24(3):473–74; What Is the Causal Interpretation of Sibling Comparison Designs?, Epidemiology 2020;31(1):75-81; Sibling-Comparison Designs, Are They Worth the Effort?, Am J Epidemiol. 2021;190(5):738-41).
As any observational study aiming to estimate causal effects, studies on medicine in pregnancy or breastfeeding should be designed within the paradigm of target trial emulation (TTE) (see Chapter 4.2.6 of this Guide). Emulating a Target Trial of Interventions Initiated During Pregnancy with Healthcare Databases: The Example of COVID-19 Vaccination (Epidemiology 2023;34(2):238-46) describes a step-by-step specification of the protocol components of a target trial and their emulation including sensitivity analyses using negative controls to evaluate the presence of confounding and, alternatively to a cohort design, a case-crossover or case-time-control design to eliminate confounding by unmeasured time-fixed factors.
Triangulation can by “integrating results from several different approaches, where each approach has different key sources of potential bias that are unrelated to each other “ further enhance the confidence in inferred causal relationships (see Triangulation in aetiological epidemiology, Int J Epidemiol. 2016;45(6):1866-86 and Chapter 6.4 of this Guide). A recent example on the use of triangulation in the assessment of safety of medication during pregnancy is Prenatal Antidepressant Exposure and the Risk of Attention-deficit/Hyperactivity Disorder in Childhood: A Cohort Study With Triangulation (Epidemiology. 2022;33(4):581-592) where additionally to the cohort analysis, a negative control analysis, a sibling analysis, and a former-user analysis were used to triangulate results.
Epidemiologically and conceptually, an ideal population in a pregnancy study should include individuals at risk for the outcome of interest at the start of eligibility. Thus, ideally, each pregnancy should be followed from pre-conception to end of pregnancy and if possible, live born infants should be followed for an appropriate period after birth to evaluate postnatal outcomes potentially related to prenatal exposure. It is even worth considering a potential impact on the next generation, such as stipulated in the Barker hypothesis, and observed, for example, after exposure in utero to environmental endocrine disruptors (Impact of intra-uterine life on future health, Ann Endocrinol (Paris) 2022;83(1):54-58).
For postnatal outcomes from e.g., interference through breastfeeding, the population at risk for adverse events resulting from such interference after birth are liveborn infants. Furthermore, because pregnancy studies deal with parent-foetus dyad, who is at risk, terminology, and possible study designs depend on the outcome of interest but always need to take into consideration the continuum of pregnancy outcomes.
Because it is challenging to capture all conceptuses in medicine safety studies in pregnancy, in practice these studies tend to be either studies of prevalence (the outcome at birth) or, slightly more informative although still with important limitations, cohort studies with left-truncation of data because cohort members are not observed from the start of the at-risk period (The curse of the perinatal epidemiologist: inferring causation amidst selection, Curr Epidemiol Rep. 2018;5(4):379-87). This implies that, with few exceptions of e.g., studies enrolling people trying to conceive (A successful implementation of e-epidemiology: the Danish pregnancy planning study ‘Snart-Gravid’, Eur J Epidemiol. 2010;25(5):297-304), studies of birth and pregnancy outcomes are cross-sectional studies, or prevalence studies (Effect measures in prevalence studies, Environ Health Perspect. 2004;112(10):1047-50), despite being commonly referred to as cohort studies in publications (Risk of adverse fetal outcomes following administration of a pandemic influenza A(H1N1) vaccine during pregnancy, JAMA 2012;308(2):165-74; Pregabalin use early in pregnancy and the risk of major congenital malformations, Neurology 2017;88(21):2020-25). As a consequence, such studies estimate prevalence as the measure of occurrence and prevalence ratios and differences as measures of effect.
The outcome spontaneous abortion has been studied using the nested case-control design with risk-set sampling by taking the case series of e.g., spontaneous abortions and sampling controls from the reconstructed set of identifiable pregnancies that are ongoing on the case occurrence (index) date (Danish group reanalyses miscarriage in NSAID users, BMJ. 2004;328(7431):109). Such design requires information on pregnancy start date for all pregnancies. In these designs, it can be challenging to ensure controls are selected from the same population as that which gave rise to the cases, if not all conceptuses are identified and, for example, only pregnancies with a recorded pregnancy outcome are available in the database. If this is not done successfully, then the control population will come from a survivors’ cohort, leading to biased risk estimates of the exposure being evaluated. Spontaneous abortions have also been studied using a cohort design with time-to-event analysis (Oseltamivir in pregnancy and birth outcomes, BMC Infect Dis. 2018;18(1):519). An important limitation of this approach is reliance on clinically recognized pregnancies, because, unless studies in pregnancy planners, most studies cannot enumerate all conceptuses at risk for death. Only when postnatal outcomes in neonates are of interest can a true cohort design be used, with liveborn infants as the population at risk, provided they are captured at birth (e.g., In utero exposure to ADHD medication and long-term offspring outcomes, Mol Psychiatry 2023). A study of postnatal outcomes that captures the subjects at the follow-up end is still a prevalence (cross-sectional) study (e.g., a study of Prenatal exposure to systemic antibacterials and overweight and obesity in Danish schoolchildren: a prevalence study, Int J Obes (Lond) 2015;39(10):1450-5).
3. Measuring medicine exposure in pregnancy
Measuring exposure and defining exposure categories as applied to pregnancy studies are similar to other pharmacoepidemiological research, including challenges of identifying over-the-counter medicine use (Medication use in pregnancy: a cross-sectional, multinational web-based study, BMJ Open 2014;4(2):e004365), defining chronic vs. occasional use (Longitudinal Methods for Modeling Exposures in Pharmacoepidemiologic Studies in Pregnancy, Epidemiol Rev. 2022;43(1):130-46) and misclassification of exposure resulting from non-adherence. However, unlike in other types of pharmacoepidemiological studies, it is not always obvious whether medicines from the same pharmacological group can be aggregated in the analysis. For studying the impact of medicine use in pregnancy, accurate exposure measurement during embryo-foetal development , i.e., the respective risk window during the pregnancy is crucial, entailing accurate measures of both pregnancy start and medicine use.
4. Measuring maternal, pregnancy, and neonatal outcomes
4.1. Different frequency of outcomes over gestational age
The frequency of many adverse pregnancy events has substantial variation over the course of pregnancy (Causal inference in studies of preterm babies: a simulation study, BJOG 2018;125(6):686-92; Two denominators for one numerator: the example of neonatal mortality, Eur J Epidemiol. 2018;33(6):523-30). For example, induced and spontaneous abortions are more frequent in early pregnancy (Incidence of early loss of pregnancy, N Engl J Med. 1988;319(4):189-94; Abortion Surveillance - United States, 2019, MMWR Surveill Summ. 2021;70(9):1-29) and stillbirths have a U-shaped distribution over gestational age later in pregnancy. Fair comparisons, in this sense, are comparisons of pregnancies at the same gestational age. This might require analysing data at various points in pregnancy (e.g., assessing the risk for foetal death by gestational week or month) in cohort studies, ensuring this is done independently of, i.e., blind to, events occurring after the index date (The fetuses-at-risk approach: survival analysis from a fetal perspective, Acta Obstet Gynecol Scand. 2018;97(4):454-65).
4.2. Safety outcomes
In observational pregnancy studies, safety outcomes are most commonly of interest. There is a set of birth and pregnancy outcomes that can be assessed, with the considerations, as above, regarding study designs, unit of observations and populations at risk.
Major congenital malformations (MCM) are often of interest. Those are often defined using the EUROCAT Methodology (Guidelines for data registration | EU RD Platform (europa.eu)). With respect to congenital malformations, it is important to acknowledge that the outcome “Any major congenital malformation” has its limitations as a single outcome because it is a composite, heterogeneous outcome. It is of limited use when studying medicine teratogenicity, especially for uncommon malformations and may lead to a ‘false sense of security’, just like there is limited rationale for aggregating all types of cancer, or all types of central nervous system disorders: any risk estimate will be biased towards the null; because of the mechanism of action of teratogens, not all organs will be affected equally. Nonetheless, because prevalence of specific birth defects identified at birth is low (e.g., spina bifida occurs at around 1:2,500 live births), and prevalence of medicine use in pregnancy is also relatively low, studies often aggregate all MCMs. Multinational studies should be used to enable adequate precision and proper causal inference based on biological mechanisms and specificity, as rarely is a medicine expected to increase the risk of all MCMs. According to the EUROCAT methodology, ascertainment of congenital malformations occurs at birth and through the first year of life and beyond, as a result of delayed detection and reporting, although of course by definition all MCMs are present at birth.
Birth outcomes typically refer to short-term (close to birth date) outcomes of a given birth relevant to the child, whereby different neonates from a multi-foetal pregnancy may be discordant with respect to the birth outcome status. Maternal outcomes typically refer to outcomes during pregnancy that affect maternal health, such as preeclampsia or diabetes. In routinely collected data, a spontaneous abortion may be recorded as a maternal diagnosis, while a stillbirth as a child’s event, whereas they both represent spontaneous pregnancy loss, defined, somewhat arbitrarily, by the gestational age at pregnancy end (Estimating the proportion of all observed birth defects occurring in pregnancies terminated by a second-trimester abortion, Epidemiology. 2014;25(6):866-71).
Table 1 summarises most common safety outcomes assessed in pharmacoepidemiological pregnancy studies, with relevant populations, study designs and suggested terminology. Definitions of gestational age, pregnancy trimesters, pregnancy loss and other pregnancy outcomes may vary as a result of differences in data availability. The Guideline on good pharmacovigilance practices (GVP) - Product- or Population-Specific Considerations III: Pregnant and breastfeeding people includes a more comprehensive list of pregnancy-related terminologies used in pharmacoepidemiological studies.
Table 1. Summary of study populations, corresponding designs, measures of occurrence, and terminology
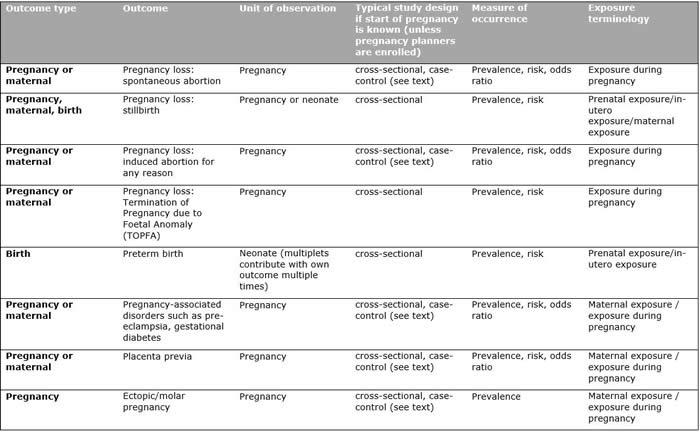
4.3. Effectiveness outcomes
As with any pharmacoepidemiological research, demonstrating effectiveness in an observational study design when it comes to medicine use in pregnancy and breastfeeding is challenging because treatment allocation is not random and the indication for prescribing is often correlated with the pregnancy outcomes of interest. However, some observational studies in this area have led to randomised controlled clinical trials to confirm or refute whether observed associations of effect were causal. Examples include folic acid, which was confirmed to reduce the risk of spina bifida, and sildenafil, for which the suggestion of a reduced risk of intra-uterine growth restriction was refuted.
Observational studies that confirmed harm, for example of pregnancy loss following influenza infection, or of lower viral load in the neonate following HIV treatment of the mother, have led to clinical guidelines recommending vaccination (influenza) or treatment (HIV) in pregnancy. Following this as well as the experience with COVID-19 vaccines and therapeutics, there is increasing recognition that effectiveness studies in pregnancy are required.
4.4. Pregnancy outcomes associated with vaccine exposure
While most concepts described in this Annex apply to any type of exposure-outcome pair, vaccine exposure in pregnancy deserves special consideration, due to:
-
its lasting effects in the mother;
-
its preventative impact against infections and diseases that may be harmful to the child;
-
the fact that vaccination may be recommended to protect the foetus or newborn;
-
the need for considering vaccination schedules and timing during pregnancy;
-
the fact that safety and effectiveness in pregnancy are rarely evaluated pre-authorisation, however, there are methodological challenges for measuring the impact of vaccination, especially on early pregnancy loss.
Specifically for vaccine exposure, a number of methodological recommendations exist, as outlined below.
The Guidance for design and analysis of observational studies of foetal and newborn outcomes following COVID-19 vaccination during pregnancy (Vaccine 2021;39(14):1882-6) provides key insights on study design, data collection, and analytical issues in COVID-19 vaccine safety studies in pregnant women, and Methodologic approaches in studies using real-world data (RWD) to measure paediatric safety and effectiveness of vaccines administered to pregnant women: A scoping review (Vaccine 2021;39(29):3814-24) describes the types of data sources that have been used in maternal immunisation studies, the methods to link maternal and infant data and estimate gestational age at time of maternal vaccination, and how exposure was documented. COVID-19 Vaccines: safety surveillance manual. Module on safety surveillance of COVID-19 vaccines in pregnant and breastfeeding women (WHO, 2021) provides guidance for the active surveillance of maternal and neonatal events, including on case definitions and methods.
In the population-based retrospective cohort study Association of SARS-CoV-2 Vaccination During Pregnancy With Pregnancy Outcomes (JAMA. 2022;327(15):1469-77), the Swedish Pregnancy Register and the Norwegian Medical Birth Registry were linked to vaccination and other registers and compared vaccinated and unvaccinated subjects, showing that vaccination in pregnancy was not associated with risks of preterm birth, stillbirth, small for gestational age and other outcomes. Spontaneous Abortion Following COVID-19 Vaccination During Pregnancy (JAMA. 2021;326(16):1629-31) applied a validated pregnancy algorithm, which incorporates diagnostic and procedure codes and EHR data, to identify and assign gestational ages for spontaneous abortions and ongoing pregnancies in the US Vaccine Safety Datalink, and analysed the odds of receiving a COVID-19 vaccine in the 28 days prior to spontaneous abortion compared with the 28 days prior to index dates for ongoing pregnancies. A potential safety issue about maternal immunisation is whether antibodies produced from pertussis vaccination during pregnancy interfere with protection during the infant schedule, an outcome referred to as blunting, as discussed in Immune interference (blunting) in the context of maternal immunization with Tdap-containing vaccines: is it a class effect? (Expert Rev Vaccines. 2020;19(4):341-352).
Pregnancy registries can be used to assess pregnancy and neonatal outcomes (see Chapter 8.3.6). Assessing the effect of vaccine on spontaneous abortion using time-dependent covariates Cox models (Pharmacoepidemiol Drug Saf. 2012;21(8):844-50) demonstrates that rates of spontaneous abortion can be severely underestimated without survival analysis techniques using time-dependent covariates to avoid immortal time bias and shows how to fit such models. Risk of miscarriage with bivalent vaccine against human papillomavirus (HPV) types 16 and 18: pooled analysis of two randomised controlled trials (BMJ. 2010; 340:c712) describes methods to calculate rates of miscarriage, addresses the lack of knowledge of time of conception during which vaccination might confer risk, and performs subgroup and sensitivity analyses. In Harmonising Immunisation Safety Assessment in Pregnancy Part I (Vaccine 2016;34 (49):5991-6110) and Part II (Vaccine 2017;35 (48), 6469-582), the Global Alignment of Immunization Safety Assessment in pregnancy (GAIA) project has provided a selection of case definitions and guidelines for the evaluation of pregnancy outcomes following immunisation. The Systematic overview of data sources for Drug Safety in pregnancy research (2016) provides an inventory of pregnancy exposure registries and alternative data sources useful to assess the safety of prenatal vaccine exposure. An illustrative, recent example of pregnancy registry is The COVID-19 Vaccines International Pregnancy Exposure Registry (C-VIPER): Protocol and Methodological Considerations (Drug Saf. 2023;46(3):297-308).
A large number of studies have generated real-world evidence on the safety and effectiveness of COVID-19 vaccination in pregnancy and on the impact of maternal immunisation on infants, yielding methodological lessons that can be applied to other types of vaccines and to pandemic preparedness. A scoping literature review identified 21 studies on the safety of COVID-19 vaccines in relation to pregnancy, delivery, and neonatal outcomes, and 12 studies on vaccine effectiveness against SARS-CoV-2 infection and COVID-19-related outcomes (Safety and effectiveness of COVID-19 maternal immunisation (EU PAS Register 47697), June 2022). Recommended informative reading includes Risk of preterm birth, small for gestational age at birth, and stillbirth after covid-19 vaccination during pregnancy: population based retrospective cohort study (BMJ. 2022;378:e071416); Maternal mRNA covid-19 vaccination during pregnancy and delta or omicron infection or hospital admission in infants: test negative design study (BMJ. 2023;380:e074035); and Maternal Vaccination and Risk of Hospitalization for Covid-19 among Infants (N Engl J Med. 2022;387(2):109-119).
5. Common confounders and biases in pregnancy studies
5.1. Confounding by indication
Treatment decisions in pregnant women are made especially thoroughly and carefully, which increases the potential of confounding by indication. To avoid or at least minimise confounding by indication, indication and severity of the underlying disease need to be taken into account. As an example, in contrast to previous studies, in Antidepressant use in pregnancy and the risk of cardiac defects (N Engl J Med. 2014;370(25):2397-407) no substantial increase in the risk of cardiac malformations attributable to antidepressant use during the first trimester was observed, when the cohort was restricted to people with depression, and propensity-score adjustment was used to control for depression severity and other potential confounders. It is recommended that researchers compare the characteristics of patients between exposure groups and perform sensitivity analyses to assess the impact of confounding by indication.
5.2. Left truncation and conditioning on pregnancy outcomes
Left-truncation refers to the situation in which data preceding the outcome of interest are not available at the time of the measurement (Conditions for bias from differential left truncation, Am J Epidemiol. 2007;165(4):444-52). Left truncation in studies that evaluate medicines in pregnancy also plays a role when early pregnancy outcomes are not captured and therefore the fact that a pregnancy existed is not known. Left truncation results in inadequate exposure assessment but because of the continuum of outcomes in pregnancy research, it can also result in the incomplete capture of study endpoints. It will result in distorted measures of association, for example when comparing people who enrol in a pregnancy registry in early pregnancy with people who enrol later (Effects of gestational age at enrollment in pregnancy exposure registries, Pharmacoepidemiol Drug Saf. 2015;24(4):343-52).
As the probability of exposure increases the longer a pregnancy lasts, shorter pregnancies will more likely be classified as unexposed. Spontaneous abortions, terminations, and outcomes associated with shorter gestational age are overrepresented in the unexposed and risk of exposure is underestimated and might even seem protective.
Not all pregnancies end in a delivery of a live born infant (Educational note: addressing special cases of bias that frequently occur in perinatal epidemiology, Int J Epidemiol. 2021;50(1):337-45). Pregnancies ending in early losses and terminations are often missed in routine healthcare data and they are routinely excluded from birth registries. Many studies therefore focus on live births (i.e., they condition on survival). Estimating the proportion of all observed birth defects occurring in pregnancies terminated by a second-trimester abortion (Epidemiology. 2014;25(6):866-71) shows that the proportion of terminated pregnancies carrying birth defects is considerably greater than the corresponding proportion for pregnancies that end as live- or stillbirths, where the proportion of terminations depends on the severity of the malformations. Thus, studies including only live births are likely to underestimate the risk of congenital malformations and early adverse pregnancy outcomes, as shown in Bias toward the null hypothesis in pregnancy drug studies that do not include data on medical terminations of pregnancy: the folic acid antagonists (J Clin Pharmacol. 2012;52(1):78-83). Methodologically, this selection bias represents conditioning on the common effect of the exposure and outcomes if exposure determines both the occurrence of the outcome of interest and survival until observable birth (A structural approach to selection bias, Epidemiology. 2004;15(5):615-25).
5.3. Immortal time bias
Immortal time bias occurs when inclusion criteria assessment and start of follow-up and exposure assessment are not aligned (see Chapter 6.1.3 of this Guide). The study Emulating Target Trials to Avoid Immortal Time Bias - An Application to Antibiotic Initiation and Preterm Delivery, Epidemiology 2023; 34 (3) 430-8 demonstrates that the previously seen protective effect of antibiotic initiation between gestational week 24 and 37 disappears once immortal time bias is avoided. This paper also describes how a sequence of target trial emulations (see Chapter 4.2.6 of this Guide) can be used to avoid immortal time bias in situations where only few persons start treatment at a certain time point, e.g. during each gestational week.
5.4. Misclassification
Sensitive exposure periods during pregnancy are usually short, and misclassification of exposure might result in considerable information bias (see Chapter 6.1.2 of this Guide). Based on prescription data, it is not possible to assess whether people started using the medicine at the dispensing date or later, discontinued treatment early once they knew they were pregnant, or took the medicine at all. Inclusion of all people with a respective dispensing overlapping the sensitive exposure period will increase sensitivity and sample size but might dilute effects due to low specificity. Linked to this, exposure ascertainment clearly needs to stop once the at-risk period for the outcome ends, because the remainder of the subsequent time is no longer ‘at risk’. For example, if spontaneous abortions are defined as foetal losses that occur up to week 20, all later time is not considered to be time ‘at risk’ for this outcome and exposure ascertainment should stop at that time.
Likewise, misclassification of outcome will also result in information bias. To address this, hypothesis driven collection of data on all relevant outcomes along the continuum of potential pregnancy outcomes is required. For longer-term outcomes such as long-term child health or neurodevelopmental disorders, adequate duration of follow-up is needed.
Sensitivity analyses should be conducted to assess the effect of potential misclassification. Such sensitivity analyses may be informed by supplementary information from surveys or interviews of a subset of the study population, for example on treatment adherence as described in Associations between socio-economic factors and the use of prescription medication during pregnancy: a population-based study among 19,874 Danish women (Eur J Clin Pharmacol. 2006;62(7):547-53).
6. Identification of pregnancies
6.1. Routinely collected data
A person typically learns that they are pregnant from a pharmacy-bought home pregnancy test. This sets in motion pregnancy-specific care, some elements of which (e.g., prenatal screening, antenatal visits, obstetric ultrasounds) are routinely recorded in some databases. In Western countries, delivery generally occurs at a hospital, and databases typically capture certain details of delivery, including mode of delivery, gestational age and birth weight, child’s sex, or admission to neonatal intensive care unit. Birth registries (such as The Nordic medical birth registers--a potential goldmine for clinical research, Acta Obstet Gynecol Scand. 2014;93(2):132-7) usually capture all live births and stillbirths after a certain gestational age (e.g., 22 weeks).
In pharmacoepidemiological research, pregnancies are identified in databases using these elements. As birth registries are considered reliable data sources for live births, studies in data sources that can be linked with birth registries often rely on them to identify pregnancies; information on the last menstruation period (LMP) and duration of gestation is obtained from the birth registry. In other databases, records on pregnancy loss, antenatal visits, deliveries and other end-of-pregnancy events are used to identify pregnancies; earlier pregnancy-related records are used to estimate the LMP or duration of gestation (Inferring pregnancy episodes and outcomes within a network of observational databases, PLoS One. 2018;13(2):e0192033; Development of an algorithm to identify pregnancy episodes in an integrated health care delivery system, Health Serv Res. 2007;42(2):908-27; Estimating the Beginning of Pregnancy in German Claims Data: Development of an Algorithm With a Focus on the Expected Delivery Date, Front Public Health. 2020;8:350; Repository of the script of the ConcePTION Algorithm for Pregnancies, ARS Toscana; COVID-19 infection and medicines in pregnancy – a multinational registry based study. Medication use in pregnant women with COVID-19: an interim report, Hurley E. et al. 2021; Zenodo 5775644).
Typically, end-of-pregnancy records, such as codes for vaginal delivery or caesarean section, are considered the most robust documentation of pregnancy, but other records can provide useful evidence. For example, the IMI ConcePTION algorithm uses all pregnancy-related records to identify pregnancies, giving precedence to those coming from birth registries and EUROCAT data tables. Another algorithm prospectively identifies codes for gestational age using the US-specific ICD-10-CM coding system in US health claims (Identification of pregnancies and infants within a US commercial healthcare administrative claims database, Pharmacoepidemiol Drug Saf. 2022 May 27). The algorithm used in UK CPRD’s Pregnancy Registers identifies pregnancies through pregnancy outcomes, but also identifies potential pregnancy episodes with unknown outcomes (Methods to generate and validate a Pregnancy Register in the UK Clinical Practice Research Datalink primary care database, Pharmacoepidemiol Drug Saf. 2019;28(7):923-33; Investigating the optimal handling of uncertain pregnancy episodes in the CPRD GOLD Pregnancy Register: a methodological study using UK primary care data, BMJ Open 2022;12(2):e055773). In German claims data, pregnancy outcomes including live births, stillbirths, induced abortions, ectopic and molar pregnancies, and spontaneous abortions can be identified based on a validated algorithm (Optimizing an algorithm for the identification and classification of pregnancy outcomes in German claims data, Pharmacoepidemiol Drug Saf. 2018 Sep;27(9):1005-1010). The expected delivery date, which can be coded in the outpatient setting can be used to validly estimate the onset of pregnancy (Estimating the Beginning of Pregnancy in German Claims Data: Development of an Algorithm With a Focus on the Expected Delivery Date, Front Public Health. 2020;8:350) and further to identify ongoing pregnancies and pregnancies with unrecorded outcome. In French claims data, an algorithm has been developed to identify and categorise pregnancy outcomes (live births, stillbirths, elective abortions, therapeutic abortions, spontaneous abortions, and ectopic pregnancies) as well as estimated pregnancy start dates, based on discharge diagnoses and medical procedures (Development of an algorithm to identify pregnancy episodes and related outcomes in health care claims databases: An application to antiepileptic drug use in 4.9 million pregnant women in France, Pharmacoepidemiol Drug Saf. 2018;27(7):763-70). While studies that focus on end-of-pregnancy events require pregnancies to be completed, drug utilisation studies, studies that assess the occurrence of pregnancy and studies that assess the prevalence of health conditions in pregnancy can benefit from the identification of ongoing pregnancies and pregnancies with unknown outcome (Investigating the optimal handling of uncertain pregnancy episodes in the CPRD GOLD Pregnancy Register: a methodological study using UK primary care data, BMJ Open 2022;12(2):e055773).
Identification of pregnancies in a database might be facilitated by use of a common data model, such as the ConcePTION (From Inception to ConcePTION: Genesis of a Network to Support Better Monitoring and Communication of Medication Safety During Pregnancy and Breastfeeding, Clin Pharmacol Ther. 2022;111(1):321-31), OMOP (Inferring pregnancy episodes and outcomes within a network of observational databases, PLoS One. 2018;13(2):e0192033) and Sentinel (Surveillance of Medication Use During Pregnancy in the Mini-Sentinel Program, Matern Child Health J. 2016;20(4):895-903) common data models. Information on the availability of pregnancies to answer a pregnancy-related research question across data sources can be facilitated through the use of catalogues like those proposed or developed for ConcePTION (Technical workshop on real-world metadata for regulatory purposes, European medicines Agency, 2021; Test report for FAIR data catalogue (1st) (D7.9), 2021, Zenodo 5829453)
Measuring exposure to medicines during breastfeeding remains a challenge in studies based on routinely collected health data.
Measuring exposure to medicines during breastfeeding remains a challenge in studies based on routinely collected health data.
6.2. Pregnancy registries
Product-specific or multiproduct pregnancy registries are among the most common surveillance methods for evaluating the impact of medicines in pregnancy; there are also disease specific registries that enable such studies. The advantage of disease-specific pregnancy registries over product-specific registries is similar to other areas of pharmacoepidemiology: they include comparator groups and the impact of the disease itself can be evaluated as well as the impact of medicine use (always bearing in mind confounding by indication). Product-specific registries tend to take a long time before generating information regarding the pregnancy outcomes of interest, often not all conceptuses are captured, and pregnancies with exposures to more than one product tend to be excluded. Considerations regarding pregnancy registries are provided in GVP Product population specific considerations III: Pregnant and breastfeeding women, Section P.III.B.4.2.1, and in Chapter 8.3.6 of this Guide.
6.3. Teratology Information Services (TIS)
According to the European Network of Teratology Information Services (ENTIS, https://www.entis-org.eu), the “main task of each TIS is to recognize and to detect risk factors with the objective of preventing birth defects. To execute this task each TIS counsels individual cases with exposure to drugs and other exogenous agents during pregnancy with respect to the risk of reproductive toxicity. The information provided is based on current scientific data, which is collected and analysed by each TIS staff.” Notably, data collected by TIS through this counselling function is not the same as formal epidemiological research on medicine safety at population level and hence, some studies based on TIS data may overestimate the drug-malformation association. For example, a TIS-based study reported a 3-fold increased risk of any major malformation associated with prenatal exposure to pregabalin (Pregnancy outcome following maternal exposure to pregabalin may call for concern, Neurology 2016;86(24):2251-7), an association that was not corroborated in a large population-based study (Pregabalin use early in pregnancy and the risk of major congenital malformations, Neurology 2017;88(21):2020-25).
6.4. Research question(s) and fit-for-purpose data sources
When studying the safety of medicines in pregnancy and/or breastfeeding, the same principles apply as in other pharmacoepidemiological studies: exposure needs to occur before the outcome, exposure and outcome must be determined with adequate precision, sample size must be such as to ensure low likelihood of Type I and Type II errors, relevant effect modifiers and confounders – including confounding by indication - must be taken into consideration, and so on. In principle, therefore, the selection of data sources is based on the same principles as in other studies. Nevertheless, all the following aspects require special consideration over and above these principles, when it comes to pregnancy studies:
-
The ability to establish which child was born to which mother (including the ability to distinguish potential mothers within the same household);
-
The duration of follow-up in the child and the ability to evaluate health and (neuro)developmental outcomes in the child;
-
Study size/precision considerations, given the need to evaluate single, or small groups of, birth defects rather than all MCMs aggregated (Planning Study Size Based on Precision Rather Than Power, Epidemiology 2018;29(5):599-603).
More often than in other areas of pharmacoepidemiology does this result in considerable added value of multi-database studies that combine different data sources, such as the EUROMEDICAT studies (combining EUROCAT data with dispensing data) and of meta-analyses in which data sources from more than one country are combined.
6.5. Pregnancy periods
EMA’s Guideline on good pharmacovigilance practices (GVP) - Product- or Population-Specific Considerations III: Pregnant and breastfeeding women lists key periods of embryo-foetal susceptibility and a pregnancy classification based on duration that can be used for protocol development.
6.6. Multinational studies
Considerations on multi-national/multi-database studies are discussed in Chapter 8 of this Guide. Multi-database studies enable study of rare exposures and outcomes (Ability of Primary Care Health Databases to Assess Medicinal Products Discussed by the European Union Pharmacovigilance Risk Assessment Committee, Clin Pharmacol Ther. 2020;107(4):957-65) and often use common data models (CDM). When choosing a CDM, main elements to consider are (i) the adaptability to a specific question; (ii) transparency to reproduce findings, assess validity, and instil confidence in findings; and (iii) ease and speed of use (Choosing Among Common Data Models for Real-World Data Analyses Fit for Making Decisions About the Effectiveness of Medical Products, Clin Pharmacol Ther. 2020;107(4):827-33). Element (i) is crucial to consider when selecting a CDM suitable for use in pregnancy and breastfeeding studies. Some key elements are needed to enable an untruncated assessment of the benefit or the risk of a drug of interest. As in all pharmacoepidemiological studies, the CDM must allow adequate capture of drug exposure and relevant outcomes. The need to observe the potential effect of a medicine taken by a mother on her child implies that the CDM must be able to adequately represent mother-child linkage. Moreover, the CDM should also allow for the storage of additional information about pregnancy, breastfeeding, or birth – e.g., gestational age, delivery data or birthweight – as these can be key covariates in analyses (Metadata-driven creation of data marts from an EAV-modeled clinical research database, Int J Med Inform. 2002;65(3):225-41). The Observational and Medical Outcomes Partnerships (OMOP) CDM and the ConcePTION CDM are two ‘person-centric’ CDMs increasingly used in the European data landscape that fulfil the previously enumerated prerequisites. The OMOP CDM is maintained by the Observational Health Data Sciences and Informatics (OHDSI) community; it implies a syntactic and a semantic harmonisation. The ConcePTION CDM (From Inception to ConcePTION: Genesis of a Network to Support Better Monitoring and Communication of Medication Safety During Pregnancy and Breastfeeding, Clin Pharmacol Ther. 2022;111(1):321-31) relies on relies on syntactic harmonisation and flexible study-specific semantic harmonisation.
Large well-designed and properly conducted population-based multinational studies are thus important to generate meaningful evidence of medicine safety in pregnancy and breastfeeding, also given the relative low prevalence of most exposures and outcomes. When combining results from different databases in multinational studies, conventional meta-analyses may not perform adequately if the number of cases in some strata is zero, as those would be removed from meta-analyses, potentially making a safe medicine appear unsafe. Alternative methods include pooling data using Mantel-Haenszel approach or individual-level data pooling, if permissible from a data protection perspective (A Population-based Study of the Safety of Gabapentin Use During Pregnancy, EUPAS 38620; Individual-based versus aggregate meta-analysis in multi-database studies of pregnancy outcomes: the Nordic example of selective serotonin reuptake inhibitors and venlafaxine in pregnancy, Pharmacoepidemiol Drug Saf. 2016;25(10):1160-69).
7. Considerations for interpretation and reporting
Interpretation of results of safety studies of medicines in pregnancy are challenging because of low prevalence of exposure and few outcome events often result in low precision of results. Importantly, systematic differences between treated and untreated are usually more severe in pregnant than in non-pregnant populations, which might lead to an overestimation of the risk even if standard methods to address confounding by indication are used. Thus, the impact of potential confounding (by indication) can be assessed, e.g., by triangulation (Triangulation in aetiological epidemiology, Int J Epidemiol. 2016;45(6):1866-1886; Prenatal Antidepressant Exposure and the Risk of Attention-deficit/Hyperactivity Disorder in Childhood: A Cohort Study With Triangulation, Epidemiology 2022;33(4):581-92; see Chapter 6.4 of this Guide). By comparison of the results from each approach, the researchers may be able to disentangle sources of bias due to potential confounding, use of sibling designs (Evaluation of nature-nurture impact on reproductive health using half-siblings, Epidemiology 1997;8(1):6-11) or negative controls (Associations of Maternal Antidepressant Use During the First Trimester of Pregnancy With Preterm Birth, Small for Gestational Age, Autism Spectrum Disorder, and Attention-Deficit/Hyperactivity Disorder in Offspring, JAMA. 2017;317(15):1553-62). Triangulation may be especially important in perinatal pharmacoepidemiology because of increased uncertainty regarding severity and impact of confounding by indication in any given study. The conjecture about the amount of residual confounding in a study may also be quantified using the E-value approach (e.g., Quantifying the impact of unmeasured confounding in observational studies with the E value, BMJ Med 2023; 2 (1):e000366).
Checklists are helpful to promote that researchers give thought to each key element or step in the study design and interpretation. It is recommended that pharmacoepidemiological studies related to the evaluation of medicines used in pregnancy heed all the elements listed in the ENCePP Checklist for Study Protocols, which is included in the ENCePP Code of Conduct. Another checklist, similar in structure, is proposed in Perinatal pharmacoepidemiology: How often are key methodological elements reported in publications? (Pharmacoepidemiol Drug Saf. 2022;31(1):61-71), which lists the following additional specific elements to be included in study protocols and reports:
-
Source of information on the beginning and end of pregnancy (to inform about the accuracy of any estimated timing of exposure relative to the outcomes critical windows);
-
Whether the study population includes multi-foetal pregnancies (to inform whether one pregnancy exposure can result in outcomes in more than one baby, and on potentially increased risks for some outcomes);
-
More than one pregnancy per person (to inform on potential intrafamily correlation);
-
Non-live births;
-
Major or minor malformations (to inform on the increased risk for some outcomes and potential for recall bias in self-reported exposures and to be explicit on outcome definitions) or neonates with chromosomal abnormalities;
-
Methods and success in matching mother and baby records or records for other family members (to inform on impact on study size and potential risk for selection bias);
-
Whether maternal or infant records are used as sources of information (to inform on potential under-ascertainment of some outcomes, for example because of insufficient duration of follow-up in the child);
-
The unit of analysis (to clarify what the denominator is and inform on potential for correlation when one maternal exposure can result in more than one offspring outcome count);
-
The gestational age at start of follow-up (to inform on left truncation and immortal-time bias) and whether intrafamily correlation is considered.
Finally, given the sensitivity of the topic, when communicating study conclusions, extra caution needs to be taken to consider the target user population. In the past, 'false alarms’ have led to unnecessary pregnancy terminations and studies with insufficient power have provided unfounded suggestions of safety. For other considerations on reporting, see Chapter 14 of this Guide.
8. Breastfeeding
For most medicines commonly used in the postnatal period, the benefits of breastfeeding for the mother and child are thought to outweigh the risks to the infant from medicine exposure through breast milk. However, to provide evidence based clinical guidelines and informative drug labels especially on the less commonly used medicines, studies assessing medicine safety in breastfed infants and human lactation studies are warranted. Passage from human blood to breastmilk is generally based on principles of passive diffusion through lipid membranes and will therefore follow a gradient from a high to a low concentration of free, unbound drug. The plasma to milk transfer depends on the pharmacokinetic (PK) properties of the substance: drugs that most easily diffuse into mother’s milk have a high concentration in maternal plasma, are fat-soluble, have a relatively low molecule weight (< 500) and a relatively low degree of protein binding in the plasma. In addition to the amount in breast milk, factors like drug toxicity and dosage, duration of treatment, as well as the infant’s age, amount of breastmilk ingested per day and the infant’s health condition need to be considered. Newborns, and premature infants in particular, have an immature liver and kidney function and thus eliminate many drugs at a considerably slower rate than older children and adults. Thus, there will be a risk of accumulation in the infant if the amount ingested though breast milk over time is larger than the infant’s capacity for metabolising and excreting the medicine. Most drugs are transferred to breast milk in amounts well below a level to exert any pharmacological effect on the infant (Drugs and Lactation Database (LactMed), Bethesda (MD): National Library of Medicine (US); 2006; Drug use and breastfeeding, Tidsskr Nor Laegeforen. 2012;132(9):1089-93). To study outcomes for exposure through breastfeeding, information on child health and neurodevelopmental outcomes in the child will need to be evaluated.
At the time of marketing authorisation, most medicines will have no data on breastfeeding, impacting the wording in the label (Guideline on Risk Assessment of Medicinal Products on Human Reproduction and Lactation: from Data to Labelling, European Medicines Agency, 2008). A review of the labels of 213 FDA-approved medicines between 2003-2012 found that there were no data on breastfeeding in 48% of labels, animal data was available in 43% of labels, whereas human breastmilk data was available in less than 5% of the labels (Trends in pregnancy labeling and data quality for US-approved pharmaceuticals, Am J Obstet Gynecol. 2014;211(6):690.e1-11). The situation in Europe is no different.
Historically, surveillance of spontaneous reports and published case reports/case series has been the main pharmacovigilance activity to assess medicine safety during breastfeeding. Fortunately, at an overall level, adverse drug reactions (ADRs) in breastfed infants are rare and usually mild (Adverse drug reactions in breastfed infants: less than imagined, Clin Pediatr (Phila). 2003;42(4):325-40; Prospective follow-up of adverse reactions in breast-fed infants exposed to maternal medication, Am J Obstet Gynecol. 1993;168(5):1393-9). Sedation, irritability, gastro-intestinal events are reported most often, with medicines acting on the central nervous system accounting for approximately half of all published suspected ADRs among breastfed infants. In approximately 80% of reported cases, the ADRs appeared in infants less than 2 months of age (Adverse drug reactions in breastfed infants: less than imagined, Clin Pediatr (Phila). 2003;42(4):325-40; Prospective follow-up of adverse reactions in breast-fed infants exposed to maternal medication, Am J Obstet Gynecol. 1993;168(5):1393-9). Published case reports of possible ADRs in breastfed infants, however, often have severe methodological limitations impairing causal inference. It is often not possible to distinguish potential drug effects from the infant’s normal state or from concurrent disease. Moreover, results are often confounded by in utero exposure. Nevertheless, case series can be valuable if performed and reported properly. When an adverse reaction is suspected in a breastfed infant, having a blood sample from the breastfed infant will improve the causality assessment.
So far, post-authorisation safety studies in breastfed children are rare. Recent EMA guidelines recommend post-authorisation studies for medicines commonly used by breastfeeding people with an unknown potential for serious adverse reactions in breastfed children. This may include a clinical lactation study and/or a prospective study following up infants exposed to a specific medicine through breast milk. Use of pregnancy registries for follow-up of breastfed infants is also possible (Guideline on good pharmacovigilance practices (GVP) - Product- or Population-Specific Considerations III: Pregnant and breastfeeding people). The Xolair® Pregnancy Registry is an example of a study providing information for the breastfeeding section of the product label (An Observational Study of the Use and Safety of Xolair® During Pregnancy, ClinicalTrials.gov, NCT00373061).
8.1. Clinical lactation studies
In general, human lactation studies are performed as a “milk only study” or a “blood & milk study”, depending on the specific medicine and study feasibility. The EMA’s Guideline on good pharmacovigilance practices (GVP) - Product- or Population-Specific Considerations III: Pregnant and breastfeeding people recommends that drug concentration levels in breast milk samples should be measured and a relative infant dose calculated to enable a risk assessment. Guidelines from FDA are also available (Clinical Lactation Studies: Considerations for Study Design Guidance for Industry, FDA 2019).
Drug concentration measurements in milk should be done at steady state, and preferably, repeated over a full dose interval. Moreover, a standardised assessment of the infant should be performed. Information on infant age is crucial and should be reported. If relevant, active drug metabolites and the maternal and infant CYP genotypes should also be included. Finally, data on the effect of the medicine on milk production or composition should be collected, if potentially clinically relevant.
Recent initiatives to improve the situation in Europe include the EU funded multinational IMI ConcePTION project. One of its aims is to establish a human milk biorepository to provide researchers, pharmaceutical industry and regulators with a system to perform human lactation studies and to establish the standards to do so. Several clinical lactation demonstration projects are ongoing (for example, Levocetirizine/cetirizine levels in human milk – an observational, clinical study among breastfeeding women, Zenodo 6345335, 2022), both as “milk only” and “blood & milk” human lactation studies. Infants are followed up and monitored for potential ADRs (incl. rash, sedation, irritability, weight gain, bleeding and gastrointestinal events). Traditional PK calculations and/or Population based PK (PopPK) modelling are being used.
Recent EMA guidelines (Guideline on good pharmacovigilance practices (GVP) - Product- or Population-Specific Considerations III: Pregnant and breastfeeding people) and EU initiatives such as IMI ConcePTION may increase post-marketing studies among breastfed infants and thus improve knowledge about medicines and breastfeeding in Europe.
9. References
A Population-based Study of the Safety of Gabapentin Use During Pregnancy. https://www.encepp.eu/encepp/viewResource.htm?id=38621
Adverse Drug Reactions in Breastfed Infants: Less Than Imagined—Philip O. Anderson, Stacey L. Pochop, Anthony S. Manoguerra, 2003. https://journals.sagepub.com/doi/10.1177/000992280304200405
An Observational Study of the Use and Safety of Xolair® During Pregnancy – ClinicalTrials.gov. https://clinicaltrials.gov/ct2/show/NCT00373061
Ananth, C. V., Platt, R. W., & Savitz, D. A. (2005). Regression models for clustered binary responses: Implications of ignoring the intracluster correlation in an analysis of perinatal mortality in twin gestations. Annals of Epidemiology, 15(4), 293–301. https://doi.org/10.1016/j.annepidem.2004.08.007
Anderson, P. O., Pochop, S. L., & Manoguerra, A. S. (2003). Adverse Drug Reactions in Breastfed Infants: Less Than Imagined. Clinical Pediatrics, 42(4), 325–340. https://doi.org/10.1177/000992280304200405
Andrade, S. E., Toh, S., Houstoun, M., Mott, K., Pitts, M., Kieswetter, C., Ceresa, C., Haffenreffer, K., & Reichman, M. E. (2016). Surveillance of Medication Use During Pregnancy in the Mini-Sentinel Program. Maternal and Child Health Journal, 20(4), 895–903. https://doi.org/10.1007/s10995-015-1878-8
Azoulay, L., Bouvattier, C., & Christin-Maitre, S. (2022). Impact of intra-uterine life on future health. Annales d’Endocrinologie, 83(1), 54–58. https://doi.org/10.1016/j.ando.2021.11.010
Bang Madsen, K., Robakis, T. K., Liu, X., Momen, N., Larsson, H., Dreier, J. W., Kildegaard, H., Groth, J. B., Newcorn, J. H., Hove Thomsen, P., Munk-Olsen, T., & Bergink, V. (2023). In utero exposure to ADHD medication and long-term offspring outcomes. Molecular Psychiatry, 28(4), Art. 4. https://doi.org/10.1038/s41380-023-01992-6
Bertoia, M. L., Phiri, K., Clifford, C. R., Doherty, M., Zhou, L., Wang, L. T., Bertoia, N. A., Wang, F. T., & Seeger, J. D. (2022). Identification of pregnancies and infants within a US commercial healthcare administrative claims database. Pharmacoepidemiology and Drug Safety, 31(8), 863–874. https://doi.org/10.1002/pds.5483
Blotière, P.-O., Weill, A., Dalichampt, M., Billionnet, C., Mezzarobba, M., Raguideau, F., Dray-Spira, R., Zureik, M., Coste, J., & Alla, F. (2018). Development of an algorithm to identify pregnancy episodes and related outcomes in health care claims databases: An application to antiepileptic drug use in 4.9 million pregnant women in France. Pharmacoepidemiology and Drug Safety, 27(7), 763–770. https://doi.org/10.1002/pds.4556
Brandt, C. A., Morse, R., Matthews, K., Sun, K., Deshpande, A. M., Gadagkar, R., Cohen, D. B., Miller, P. L., & Nadkarni, P. M. (2002). Metadata-driven creation of data marts from an EAV-modeled clinical research database. International Journal of Medical Informatics, 65(3), 225–241. https://doi.org/10.1016/S1386-5056(02)00047-3
Campbell, J., Bhaskaran, K., Thomas, S., Williams, R., McDonald, H. I., & Minassian, C. (2022). Investigating the optimal handling of uncertain pregnancy episodes in the CPRD GOLD Pregnancy Register: A methodological study using UK primary care data. BMJ Open, 12(2), e055773. https://doi.org/10.1136/bmjopen-2021-055773
Caniglia, E. C., Zash, R., Fennell, C., Diseko, M., Mayondi, G., Heintz, J., Mmalane, M., Makhema, J., Lockman, S., Mumford, S. L., Murray, E. J., Hernández-Díaz, S., & Shapiro, R. (2023). Emulating Target Trials to Avoid Immortal Time Bias – An Application to Antibiotic Initiation and Preterm Delivery. Epidemiology, 34(3), 430. https://doi.org/10.1097/EDE.0000000000001601
Clinical Lactation Studies: Considerations for Study Design Guidance for Industry. (2019). Clinical Lactation. https://www.fda.gov/media/124749/download
Code of Conduct. https://www.encepp.eu/code_of_conduct/index.shtml
ConcePTION. www.imi-conception.eu
ConcePTION Algorithm Pregnancies. (2023). [R]. Agenzia Regionale di Sanità della Toscana. https://github.com/ARS-toscana/ConcePTIONAlgorithmPregnancies (Original work published 2021)
COVID-19 Vaccines: Safety surveillance manual. Module on safety surveillance of COVID-19 vaccines in pregnant and breastfeeding women. https://www.who.int/publications-detail-redirect/WHO-MHP-RPQ-PVG-2021.1
Drugs and Lactation Database (LactMed®). (2006). National Institute of Child Health and Human Development.
Ehrenstein, V., Kristensen, N. R., Monz, B. U., Clinch, B., Kenwright, A., & Sørensen, H. T. (2018). Oseltamivir in pregnancy and birth outcomes. BMC Infectious Diseases, 18(1), 519. https://doi.org/10.1186/s12879-018-3423-z
Good pharmacovigilance practices. European Medicines Agency. https://www.ema.europa.eu/en/human-regulatory/post-authorisation/pharmacovigilance/good-pharmacovigilance-practices
Technical workshop on real-world metadata for regulatory purposes European Medicines Agency. https://www.ema.europa.eu/en/events/technical-workshop-real-world-metadata-regulatory-purposes
ENCePP Checklist for Study Protocols. ENCePP. https://www.encepp.eu/standards_and_guidances/checkListProtocols.shtml
ENTIS. (2023, June 21). https://www.entis-org.eu/
Esen, B. Ö., Ehrenstein, V., Sørensen, H. T., Laugesen, K., & Pedersen, L. (2022). Prenatal Antidepressant Exposure and the Risk of Attention-deficit/Hyperactivity Disorder in Childhood: A Cohort Study With Triangulation. Epidemiology, 33(4), 581. https://doi.org/10.1097/EDE.0000000000001494
Estimating the Proportion of All Observed Birth Defects Occu... : Epidemiology. https://journals.lww.com/epidem/Fulltext/2014/11000/Estimating_the_Proportion_of_All_Observed_Birth.12.aspx
European Platform on Rare Disease Registration. https://eu-rd-platform.jrc.ec.europa.eu
Fell, D. B., Dimanlig-Cruz, S., Regan, A. K., Håberg, S. E., Gravel, C. A., Oakley, L., Alton, G. D., Török, E., Dhinsa, T., Shah, P. S., Wilson, K., Sprague, A. E., El-Chaâr, D., Walker, M. C., Barrett, J., Okun, N., Buchan, S. A., Kwong, J. C., Wilson, S. E., … Dougan, S. D. (2022). Risk of preterm birth, small for gestational age at birth, and stillbirth after covid-19 vaccination during pregnancy: Population based retrospective cohort study. BMJ (Clinical Research Ed.), 378, e071416. https://doi.org/10.1136/bmj-2022-071416
Fell, D. B., Dimitris, M. C., Hutcheon, J. A., Ortiz, J. R., Platt, R. W., Regan, A. K., & Savitz, D. A. (2021). Guidance for design and analysis of observational studies of fetal and newborn outcomes following COVID-19 vaccination during pregnancy. Vaccine, 39(14), 1882–1886. https://doi.org/10.1016/j.vaccine.2021.02.070
Flynn, R., Hedenmalm, K., Murray-Thomas, T., Pacurariu, A., Arlett, P., Shepherd, H., Myles, P., & Kurz, X. (2020). Ability of Primary Care Health Databases to Assess Medicinal Products Discussed by the European Union Pharmacovigilance Risk Assessment Committee. Clinical Pharmacology & Therapeutics, 107(4), 957–965. https://doi.org/10.1002/cpt.1775
Frisell, T. (2021). Invited Commentary: Sibling-Comparison Designs, Are They Worth the Effort? American Journal of Epidemiology, 190(5), 738–741. https://doi.org/10.1093/aje/kwaa183
Guideline on Risk Assessment of Medicinal Products on Human Reproduction and Lactation: From Data to Labelling. https://www.ema.europa.eu/en/documents/scientific-guideline/guideline-risk-assessment-medicinal-products-human-reproduction-lactation-data-labelling_en.pdf
Halasa, N. B., Olson, S. M., Staat, M. A., Newhams, M. M., Price, A. M., Pannaraj, P. S., Boom, J. A., Sahni, L. C., Chiotos, K., Cameron, M. A., Bline, K. E., Hobbs, C. V., Maddux, A. B., Coates, B. M., Michelson, K. N., Heidemann, S. M., Irby, K., Nofziger, R. A., Mack, E. H., … Overcoming Covid-19 Investigators. (2022). Maternal Vaccination and Risk of Hospitalization for Covid-19 among Infants. The New England Journal of Medicine, 387(2), 109–119. https://doi.org/10.1056/NEJMoa2204399
Harmon, Q. E., Basso, O., Weinberg, C. R., & Wilcox, A. J. (2018). Two denominators for one numerator: The example of neonatal mortality. European Journal of Epidemiology, 33(6), 523–530. https://doi.org/10.1007/s10654-018-0373-0
Harmonising Immunisation Safety Assessment in Pregnancy Part I. (2016). Vaccine, 34(49), Art. 49. https://doi.org/10.1016/S0264-410X(16)31046-5
Harmonising Immunisation Safety Assessment in Pregnancy Part II. (2017). Vaccine, 35(48, Part A), Art. 48, Part A. https://doi.org/10.1016/S0264-410X(17)31553-0
Hernán, M. A., Hernández-Díaz, S., & Robins, J. M. (2004). A Structural Approach to Selection Bias. Epidemiology, 15(5), 615. https://doi.org/10.1097/01.ede.0000135174.63482.43
Hernández-Díaz, S., Huybrechts, K. F., Chiu, Y.-H., Yland, J. J., Bateman, B. T., & Hernán, M. A. (2023). Emulating a Target Trial of Interventions Initiated During Pregnancy with Healthcare Databases: The Example of COVID-19 Vaccination. Epidemiology, 34(2), 238. https://doi.org/10.1097/EDE.0000000000001562
Hornbrook, M. C., Whitlock, E. P., Berg, C. J., Callaghan, W. M., Bachman, D. J., Gold, R., Bruce, F. C., Dietz, P. M., & Williams, S. B. (2007). Development of an Algorithm to Identify Pregnancy Episodes in an Integrated Health Care Delivery System. Health Services Research, 42(2), 908–927. https://doi.org/10.1111/j.1475-6773.2006.00635.x
Howards, P. P., Hertz-Picciotto, I., & Poole, C. (2007). Conditions for Bias from Differential Left Truncation. American Journal of Epidemiology, 165(4), 444–452. https://doi.org/10.1093/aje/kwk027
Hurley, E., Sturkenboom, M., Poblador-Plou, B., Sanfelix-Gimeno, G., Sanchez, F., Hurtado, I., Rodriguez, C., Jordan, S., Thayer, D., Scanlon, I., Bartolini, C., Limoncella, G., Gini, R., & Nordeng, H. (2021). COVID-19 infection and medicines in pregnancy – a multinational registry based study Medication use in pregnant women with COVID-19: An interim report. Zenodo. https://doi.org/10.5281/zenodo.5775644
Huybrechts, K. F., Bateman, B. T., & Hernández-Díaz, S. (2019). Use of real-world evidence from healthcare utilization data to evaluate drug safety during pregnancy. Pharmacoepidemiology and Drug Safety, 28(7), 906–922. https://doi.org/10.1002/pds.4789
Huybrechts, K. F., Mikkelsen, E. M., Christensen, T., Riis, A. H., Hatch, E. E., Wise, L. A., Sørensen, H. T., & Rothman, K. J. (2010). A successful implementation of e-epidemiology: The Danish pregnancy planning study ‘Snart-Gravid’. European Journal of Epidemiology, 25(5), 297–304. https://doi.org/10.1007/s10654-010-9431-y
Huybrechts, K. F., Palmsten, K., Avorn, J., Cohen, L. S., Holmes, L. B., Franklin, J. M., Mogun, H., Levin, R., Kowal, M., Setoguchi, S., & Hernández-Díaz, S. (2014). Antidepressant Use in Pregnancy and the Risk of Cardiac Defects. New England Journal of Medicine, 370(25), 2397–2407. https://doi.org/10.1056/NEJMoa1312828
Informatics, O. H. D. S. and. The Book of OHDSI. https://ohdsi.github.io/TheBookOfOhdsi/
Ito, S., Blajchman, A., Stephenson, M., Eliopoulos, C., & Koren, G. (1993a). Prospective follow-up of adverse reactions in breast-fed infants exposed to maternal medication. American Journal of Obstetrics and Gynecology, 168(5), 1393–1399. https://doi.org/10.1016/S0002-9378(11)90771-6
Ito, S., Blajchman, A., Stephenson, M., Eliopoulos, C., & Koren, G. (1993b). Prospective follow-up of adverse reactions in breast-fed infants exposed to maternal medication. American Journal of Obstetrics and Gynecology, 168(5), 1393–1399. https://doi.org/10.1016/S0002-9378(11)90771-6
Jorgensen, S. C. J., Hernandez, A., Fell, D. B., Austin, P. C., D’Souza, R., Guttmann, A., Brown, K. A., Buchan, S. A., Gubbay, J. B., Nasreen, S., Schwartz, K. L., Tadrous, M., Wilson, K., Kwong, J. C., & Canadian Immunization Research Network (CIRN) Provincial Collaborative Network (PCN) Investigators. (2023). Maternal mRNA covid-19 vaccination during pregnancy and delta or omicron infection or hospital admission in infants: Test negative design study. BMJ (Clinical Research Ed.), 380, e074035. https://doi.org/10.1136/bmj-2022-074035
Joseph, K. s., & Kramer, M. S. (2018). The fetuses-at-risk approach: Survival analysis from a fetal perspective. Acta Obstetricia et Gynecologica Scandinavica, 97(4), 454–465. https://doi.org/10.1111/aogs.13194
Kandeil, W., Savic, M., Ceregido, M. A., Guignard, A., Kuznetsova, A., & Mukherjee, P. (2020). Immune interference (blunting) in the context of maternal immunization with Tdap-containing vaccines: Is it a class effect? Expert Review of Vaccines, 19(4), 341–352. https://doi.org/10.1080/14760584.2020.1749597
Keyes, K. M., Smith, G. D., & Susser, E. (2013). On Sibling Designs. Epidemiology (Cambridge, Mass.), 24(3), 473–474. https://doi.org/10.1097/EDE.0b013e31828c7381
Kharbanda, E. O., Haapala, J., DeSilva, M., Vazquez-Benitez, G., Vesco, K. K., Naleway, A. L., & Lipkind, H. S. (2021). Spontaneous Abortion Following COVID-19 Vaccination During Pregnancy. JAMA, 326(16), 1629–1631. https://doi.org/10.1001/jama.2021.15494
Kortsmit, K. (2021). Abortion Surveillance—United States, 2019. MMWR. Surveillance Summaries, 70. https://doi.org/10.15585/mmwr.ss7009a1
Langhoff-Roos, J., Krebs, L., Klungsøyr, K., Bjarnadottir, R. I., Källén, K., Tapper, A.-M., Jakobsson, M., Børdahl, P. E., Lindqvist, P. G., Gottvall, K., Colmorn, L. B., & Gissler, M. (2014). The Nordic medical birth registers – a potential goldmine for clinical research. Acta Obstetricia et Gynecologica Scandinavica, 93(2), 132–137. https://doi.org/10.1111/aogs.12302
Lasky, T., McMahon, A. W., Hua, W., & Forshee, R. (2021). Methodologic approaches in studies using real-world data (RWD) to measure pediatric safety and effectiveness of vaccines administered to pregnant women: A scoping review. Vaccine, 39(29), 3814–3824. https://doi.org/10.1016/j.vaccine.2021.05.071
Lawlor, D. A., Tilling, K., & Davey Smith, G. (2016). Triangulation in aetiological epidemiology. International Journal of Epidemiology, 45(6), 1866–1886. https://doi.org/10.1093/ije/dyw314
Levy, A., Matok, I., Gorodischer, R., Sherf, M., Wiznitzer, A., Uziel, E., & Koren, G. (2012). Bias Toward the Null Hypothesis in Pregnancy Drug Studies That Do Not Include Data on Medical Terminations of Pregnancy: The Folic Acid Antagonists. The Journal of Clinical Pharmacology, 52(1), 78–83. https://doi.org/10.1177/0091270010390806
Lupattelli, A., Spigset, O., Twigg, M. J., Zagorodnikova, K., Mårdby, A. C., Moretti, M. E., Drozd, M., Panchaud, A., Hämeen-Anttila, K., Rieutord, A., Juraski, R. G., Odalovic, M., Kennedy, D., Rudolf, G., Juch, H., Passier, A., Björnsdóttir, I., & Nordeng, H. (2014). Medication use in pregnancy: A cross-sectional, multinational web-based study. BMJ Open, 4(2), e004365. https://doi.org/10.1136/bmjopen-2013-004365
Magnus, M. C., Örtqvist, A. K., Dahlqwist, E., Ljung, R., Skår, F., Oakley, L., Macsali, F., Pasternak, B., Gjessing, H. K., Håberg, S. E., & Stephansson, O. (2022). Association of SARS-CoV-2 Vaccination During Pregnancy With Pregnancy Outcomes. JAMA, 327(15), 1469–1477. https://doi.org/10.1001/jama.2022.3271
Margulis, A. V., Kawai, A. T., Anthony, M. S., & Rivero-Ferrer, E. (2022). Perinatal pharmacoepidemiology: How often are key methodological elements reported in publications? Pharmacoepidemiology and Drug Safety, 31(1), 61–71. https://doi.org/10.1002/pds.5353
Margulis, A. V., Mittleman, M. A., Glynn, R. J., Holmes, L. B., & Hernández-Díaz, S. (2015). Effects of gestational age at enrollment in pregnancy exposure registries. Pharmacoepidemiology and Drug Safety, 24(4), 343–352. https://doi.org/10.1002/pds.3731
Matcho, A., Ryan, P., Fife, D., Gifkins, D., Knoll, C., & Friedman, A. (2018). Inferring pregnancy episodes and outcomes within a network of observational databases. PLOS ONE, 13(2), e0192033. https://doi.org/10.1371/journal.pone.0192033
Mazer-Amirshahi, M., Samiee-Zafarghandy, S., Gray, G., & van den Anker, J. N. (2014). Trends in pregnancy labeling and data quality for US-approved pharmaceuticals. American Journal of Obstetrics and Gynecology, 211(6), 690.e1-690.e11. https://doi.org/10.1016/j.ajog.2014.06.013
Minassian, C., Williams, R., Meeraus, W. H., Smeeth, L., Campbell, O. M. R., & Thomas, S. L. (2019). Methods to generate and validate a Pregnancy Register in the UK Clinical Practice Research Datalink primary care database. Pharmacoepidemiology and Drug Safety, 28(7), 923–933. https://doi.org/10.1002/pds.4811
Mor, A., Antonsen, S., Kahlert, J., Holsteen, V., Jørgensen, S., Holm-Pedersen, J., Sørensen, H. T., Pedersen, O., & Ehrenstein, V. (2015). Prenatal exposure to systemic antibacterials and overweight and obesity in Danish schoolchildren: A prevalence study. International Journal of Obesity, 39(10), Art. 10. https://doi.org/10.1038/ijo.2015.129
Neophytou, A. M., Kioumourtzoglou, M.-A., Goin, D. E., Darwin, K. C., & Casey, J. A. (2020). Educational note: Addressing special cases of bias that frequently occur in perinatal epidemiology. International Journal of Epidemiology, 50(1), 337–345. https://doi.org/10.1093/ije/dyaa252
Nielsen, G. L., Skriver, M. V., Pedersen, L., & S⊘rensen, H. T. (2004). Danish group reanalyses miscarriage in NSAID users. BMJ, 328(7431), 109. https://doi.org/10.1136/bmj.328.7431.109
Nordeng, H. (2022). Levocetirizine/cetirizine levels in human milk – an observational, clinical study among breastfeeding women. https://doi.org/10.5281/zenodo.6345336
Nordeng, H., Havnen, G. C., & Spigset, O. (2012). Legemiddelbruk ved amming. Tidsskrift for Den norske legeforening. https://tidsskriftet.no/2012/05/oversiktsartikkel/legemiddelbruk-ved-amming
Olesen, C., Thrane, N., Henriksen, T. B., Ehrenstein, V., & Olsen, J. (2006). Associations between socio-economic factors and the use of prescription medication during pregnancy. European Journal of Clinical Pharmacology, 62(7), 547–553. https://doi.org/10.1007/s00228-006-0119-x
Pariente, G., Leibson, T., Carls, A., Adams-Webber, T., Ito, S., & Koren, G. (2016). Pregnancy-Associated Changes in Pharmacokinetics: A Systematic Review. PLOS Medicine, 13(11), e1002160. https://doi.org/10.1371/journal.pmed.1002160
Pasternak, B., Svanström, H., Mølgaard-Nielsen, D., Krause, T. G., Emborg, H.-D., Melbye, M., & Hviid, A. (2012). Risk of Adverse Fetal Outcomes Following Administration of a Pandemic Influenza A(H1N1) Vaccine During Pregnancy. JAMA, 308(2), 165–174. https://doi.org/10.1001/jama.2012.6131
Patorno, E., Bateman, B. T., Huybrechts, K. F., MacDonald, S. C., Cohen, J. M., Desai, R. J., Panchaud, A., Mogun, H., Pennell, P. B., & Hernandez-Diaz, S. (2017a). Pregabalin use early in pregnancy and the risk of major congenital malformations. Neurology, 88(21), 2020–2025. https://doi.org/10.1212/WNL.0000000000003959
Patorno, E., Bateman, B. T., Huybrechts, K. F., MacDonald, S. C., Cohen, J. M., Desai, R. J., Panchaud, A., Mogun, H., Pennell, P. B., & Hernandez-Diaz, S. (2017b). Pregabalin use early in pregnancy and the risk of major congenital malformations. Neurology, 88(21), 2020–2025. https://doi.org/10.1212/WNL.0000000000003959
Pearce, N. (2004). Effect Measures in Prevalence Studies. Environmental Health Perspectives, 112(10), 1047–1050. https://doi.org/10.1289/ehp.6927
Petersen, A. H., & Lange, T. (2020). What Is the Causal Interpretation of Sibling Comparison Designs? Epidemiology (Cambridge, Mass.), 31(1), 75–81. https://doi.org/10.1097/EDE.0000000000001108
Petersen, I., Gilbert, R. E., Evans, S. J. W., Man, S.-L., & Nazareth, I. (2011). Pregnancy as a Major Determinant for Discontinuation of Antidepressants: An Analysis of Data From The Health Improvement Network. The Journal of Clinical Psychiatry, 72(7), 15209. https://doi.org/10.4088/JCP.10m06090blu
Prevalence of maternal chronic diseases during pregnancy – a nationwide population based study from 1989 to 2013—Jølving—2016—Acta Obstetricia et Gynecologica Scandinavica—Wiley Online Library. https://obgyn.onlinelibrary.wiley.com/doi/10.1111/aogs.13007
Quantifying the impact of unmeasured confounding in observational studies with the E value | BMJ Medicine. https://bmjmedicine.bmj.com/content/2/1/e000366
Rahu, M. (1997). Building Bridges across Diversity. Epidemiology, 8(1), 1. https://journals.lww.com/epidem/Citation/1997/01000/Building_Bridges_across_Diversity.1.aspx
Rothman, K. J., & Greenland, S. (2018). Planning Study Size Based on Precision Rather Than Power. Epidemiology, 29(5), 599. https://doi.org/10.1097/EDE.0000000000000876
Safety and effectiveness of COVID-19 maternal immunisation: An update of available evidence. https://www.encepp.eu/encepp/viewResource.htm?id=47698
Schink, T., Wentzell, N., Dathe, K., Onken, M., & Haug, U. (2020). Estimating the Beginning of Pregnancy in German Claims Data: Development of an Algorithm With a Focus on the Expected Delivery Date. Frontiers in Public Health, 8. https://www.frontiersin.org/articles/10.3389/fpubh.2020.00350
Schneeweiss, S., Brown, J. S., Bate, A., Trifirò, G., & Bartels, D. B. (2020). Choosing Among Common Data Models for Real-World Data Analyses Fit for Making Decisions About the Effectiveness of Medical Products. Clinical Pharmacology & Therapeutics, 107(4), 827–833. https://doi.org/10.1002/cpt.1577
Selmer, R., Haglund, B., Furu, K., Andersen, M., Nørgaard, M., Zoëga, H., & Kieler, H. (2016). Individual-based versus aggregate meta-analysis in multi-database studies of pregnancy outcomes: The Nordic example of selective serotonin reuptake inhibitors and venlafaxine in pregnancy. Pharmacoepidemiology and Drug Safety, 25(10), 1160–1169. https://doi.org/10.1002/pds.4033
SNOWDEN, J. M., & BASSO, O. (2018). Causal inference in studies of preterm babies: A simulation study. BJOG : An International Journal of Obstetrics and Gynaecology, 125(6), 686–692. https://doi.org/10.1111/1471-0528.14942
Snowden, J. M., Bovbjerg, M. L., Dissanayake, M., & Basso, O. (2018). The Curse of the Perinatal Epidemiologist: Inferring Causation Amidst Selection. Current Epidemiology Reports, 5(4), 379–387. https://doi.org/10.1007/s40471-018-0172-x
Sujan, A. C., Rickert, M. E., Öberg, A. S., Quinn, P. D., Hernández-Díaz, S., Almqvist, C., Lichtenstein, P., Larsson, H., & D’Onofrio, B. M. (2017). Associations of Maternal Antidepressant Use During the First Trimester of Pregnancy With Preterm Birth, Small for Gestational Age, Autism Spectrum Disorder, and Attention-Deficit/Hyperactivity Disorder in Offspring. JAMA, 317(15), 1553–1562. https://doi.org/10.1001/jama.2017.3413
Svensson, E., Ehrenstein, V., Nørgaard, M., Bakketeig, L. S., Rothman, K. J., Toft Sørensen, H., & Pedersen, L. (2014). Estimating the Proportion of All Observed Birth Defects Occurring in Pregnancies Terminated by a Second-trimester Abortion. Epidemiology, 25(6), 866. https://doi.org/10.1097/EDE.0000000000000163
Swertz, M., Hyde, E., Cunnington, M., & Gini, R. (2021). Test report for FAIR data catalogue (1st) (D7.9). https://zenodo.org/record/5829454
Thurin, N. H., Pajouheshnia, R., Roberto, G., Dodd, C., Hyeraci, G., Bartolini, C., Paoletti, O., Nordeng, H., Wallach-Kildemoes, H., Ehrenstein, V., Dudukina, E., MacDonald, T., De Paoli, G., Loane, M., Damase-Michel, C., Beau, A.-B., Droz-Perroteau, C., Lassalle, R., Bergman, J., … Gini, R. (2022a). From Inception to ConcePTION: Genesis of a Network to Support Better Monitoring and Communication of Medication Safety During Pregnancy and Breastfeeding. Clinical Pharmacology & Therapeutics, 111(1), 321–331. https://doi.org/10.1002/cpt.2476
Thurin, N. H., Pajouheshnia, R., Roberto, G., Dodd, C., Hyeraci, G., Bartolini, C., Paoletti, O., Nordeng, H., Wallach-Kildemoes, H., Ehrenstein, V., Dudukina, E., MacDonald, T., De Paoli, G., Loane, M., Damase-Michel, C., Beau, A.-B., Droz-Perroteau, C., Lassalle, R., Bergman, J., … Gini, R. (2022b). From Inception to ConcePTION: Genesis of a Network to Support Better Monitoring and Communication of Medication Safety During Pregnancy and Breastfeeding. Clinical Pharmacology & Therapeutics, 111(1), 321–331. https://doi.org/10.1002/cpt.2476
Wacholder, S., Chen, B. E., Wilcox, A., Macones, G., Gonzalez, P., Befano, B., Hildesheim, A., Rodríguez, A. C., Solomon, D., Herrero, R., & Schiffman, M. (2010). Risk of miscarriage with bivalent vaccine against human papillomavirus (HPV) types 16 and 18: Pooled analysis of two randomised controlled trials. BMJ, 340, c712. https://doi.org/10.1136/bmj.c712
Wentzell, N., Schink, T., Haug, U., Ulrich, S., Niemeyer, M., & Mikolajczyk, R. (2018). Optimizing an algorithm for the identification and classification of pregnancy outcomes in German claims data. Pharmacoepidemiology and Drug Safety, 27(9), 1005–1010. https://doi.org/10.1002/pds.4588
Wilcox, A. J., Weinberg, C. R., O’Connor, J. F., Baird, D. D., Schlatterer, J. P., Canfield, R. E., Armstrong, E. G., & Nisula, B. C. (1988). Incidence of Early Loss of Pregnancy. New England Journal of Medicine, 319(4), 189–194. https://doi.org/10.1056/NEJM198807283190401
Winterfeld, U., Merlob, P., Baud, D., Rousson, V., Panchaud, A., Rothuizen, L. E., Bernard, N., Vial, T., Yates, L. M., Pistelli, A., Ellfolk, M., Eleftheriou, G., Vries, L. C. de, Jonville-Bera, A.-P., Kadioglu, M., Biollaz, J., & Buclin, T. (2016). Pregnancy outcome following maternal exposure to pregabalin may call for concern. Neurology, 86(24), 2251–2257. https://doi.org/10.1212/WNL.0000000000002767
Women in the EU are having their first child later. https://ec.europa.eu/eurostat/web/products-eurostat-news/-/ddn-20210224-1
Wood, M. E., Lupattelli, A., Palmsten, K., Bandoli, G., Hurault-Delarue, C., Damase-Michel, C., Chambers, C. D., Nordeng, H. M. E., & van Gelder, M. M. H. J. (2021). Longitudinal Methods for Modeling Exposures in Pharmacoepidemiologic Studies in Pregnancy. Epidemiologic Reviews, 43(1), 130–146. https://doi.org/10.1093/epirev/mxab002
Wyszynski, D. F., Bhattacharya, M., Martínez-Pérez, O., Scialli, A. R., Tassinari, M., Bar-Zeev, N., Renz, C., & Hernández-Díaz, S. (2023). The COVID-19 Vaccines International Pregnancy Exposure Registry (C-VIPER): Protocol and Methodological Considerations. Drug Safety, 46(3), 297–308. https://doi.org/10.1007/s40264-022-01271-3
Xu, R., Luo, Y., & Chambers, C. (2012). Assessing the effect of vaccine on spontaneous abortion using time-dependent covariates Cox models. Pharmacoepidemiology and Drug Safety, 21(8), 844–850. https://doi.org/10.1002/pds.3301